LLM FINE-TUNING SERVICES BY FOLIO3 AI
Optimize Your AI Models to Drive Business Excellence
Maximize the potential of large language models (LLMs) with our comprehensive fine-tuning services tailored specifically to your unique business needs.
Highly Customized AI Models Tailored to Your Business With LLM Fine Tuning Services
Fine-tuning is the process of adapting pre-trained language models to specific business tasks by refining their parameters, datasets, and response mechanisms. This means highly customized AI models for businesses that better understand your industry, deliver more accurate results and integrate easily into your operations. LLM fine-tuning ensures that AI systems not only speak your language but also think in terms of your specific business goals and challenges.
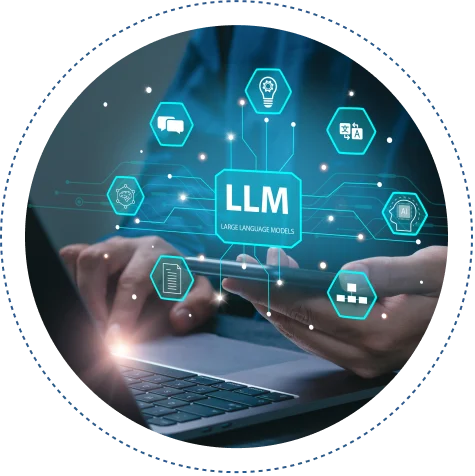
Our Expertise in LLM Fine-Tuning
At Folio3 AI, we offer a broad range of services to fine-tune your LLM models, making them more relevant and effective for your business.
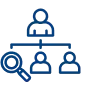
LLM Consultation
we assess your business needs, challenges, and goals. We dive deep into understanding your existing infrastructure, the data you work with, and the specific tasks you aim to optimize to begin the process. This detailed evaluation allows us to identify the most suitable LLM models and fine-tuning strategies that will deliver the highest impact on your business performance.
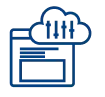
Custom Model Training
Based on the insights gathered during the consultation, we proceed to select and customize LLMs tailored to your industry. This involves choosing the right pre-trained models and adjusting them to your unique requirements through specialized training. Our custom model training focuses on optimizing the model's performance for the specific tasks it will perform within your organization, ensuring that it delivers accurate, relevant, and efficient results.
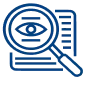
Thorough Testing & Evaluation
We also test and evaluate the fine-tuned models to ensure they meet your business standards. Our testing phase includes stress testing, performance benchmarking, and scenario-based evaluations to simulate real-world applications. We provide a detailed report on the model's performance, highlighting areas of strength and any potential improvements needed to fully integrate the model into your operations.
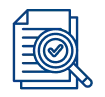
Data Selection & Preparation
The quality of data used for fine-tuning is critical to the success of the model. We curate and refine datasets that align with your business objectives. This involves cleaning, organizing, and formatting data to ensure that the model is trained on the most relevant and accurate information. Our data preparation process is designed to enhance the relevancy and performance of the model, resulting in more precise outputs that meet your specific business needs.
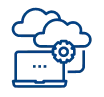
LLM Model Integration
Once fine-tuning is complete, we integrate the optimized model into your existing systems. Our integration process is designed to ensure that the new model works with your current technology stack, minimizing disruptions and maximizing the benefits of AI. We provide ongoing support during the integration phase, ensuring that the transition is smooth and the model is performing as intended in its live environment.
The LLM Fine-Tuning Process
How We Fine-Tune Your Models
At Folio3 AI, we follow a structured process to ensure your LLM models are perfectly tuned:
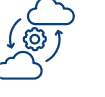
Data Processing
Aggregate and clean data from multiple sources to create a unified, relevant dataset.
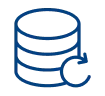
Model Selection
Choose the optimal model based on task complexity and business needs.
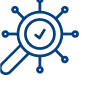
LLM Parameters Identification
Identify key parameters such as learning rate and batch size for fine-tuning.
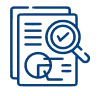
Model Testing & Refining
Test the model’s performance, iterating until it meets the desired accuracy and efficiency.
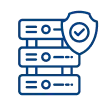
Deployment & Monitoring
Integrate the fine-tuned model into your system and monitor its performance for continuous improvements.
Why Choose Folio3 AI for Your LLM Fine-Tuning Services
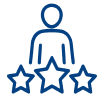
15+ Years of Proven Expertise
With over 15 years of experience in AI and ML development, Folio3 AI has a proven track record of delivering successful, high-impact projects.
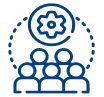
1000+ Enterprise-Level Clients
Over the last 15 years, we've built an extensive client base of delighted customers!
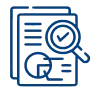
Technical Expertise
Leverage cutting-edge technologies and best-in-class resources to fine-tune your models.
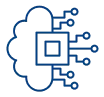
Ethical AI Development
We ensure secure, ethical, and bias-free AI solutions that align with your values.
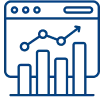
Industry-Specific Solutions
Benefit from models finely tuned to the needs of your specific industry.
FAQs
What is LLM Fine Tuning?
LLM Fine Tuning involves adapting a pre-trained Large Language Model (LLM) to a specific task or domain by training it on a smaller, specialized dataset relevant to the intended application.
What is the difference between LLM Fine Tuning and LLM Model Development?
Fine tuning adjusts an existing pre-trained model for specific needs, while model development involves building a new language model from scratch, requiring more data and computational resources.
Why Does a Business Need LLM Fine Tuning Services?
Businesses need LLM Fine Tuning to customize AI models for industry-specific language, improving the model’s relevance, accuracy, and efficiency in meeting their unique requirements.
What are the benefits of LLM Fine Tuning Service?
LLM Fine Tuning enhances model performance by increasing accuracy, reducing training time, lowering costs, and ensuring the AI system better understands domain-specific contexts and jargon.
What is Supervised Fine Tuning?
Supervised Fine Tuning is the process of improving a pre-trained AI model by training it with labeled data, where each input has a known, correct output. This helps the model learn to perform specific tasks more accurately, like understanding certain types of questions or recognizing patterns in a particular field.