Big data has always been invaluable in business. It analyzes a large chunk of information, whether it’s customer behavior, social media trends, or sensor data. It then reveals trends and patterns that would only be possible to see with smaller datasets.
This data will increase rapidly in 2024. As AI rules the world, sensors, facial recognition, or similar technologies also generate massive bundles of data. This data needs to be used effectively to provide insight. Therefore, predictive analytics is being used to help predict future market trends so businesses can hit their targets.
Here’s how it works: Predictive analytics uses data analysis techniques to forecast future outcomes. For example, it uses past sales data to figure out how much stock you’ll need next month. This isn’t just guesswork; it’s about using solid data to make smarter decisions.
However, predictive analytics has the power to enrich businesses. Such as
- Reduced Costs: Predict equipment failures before they happen, preventing costly downtime.
- Increased Sales: Target marketing campaigns to the right customers at the right time, boosting sales.
- Improved Customer Experience: Anticipate customer needs and personalize their experience.
Now that you see why predicting data is important, it’s time to look into some examples to get a clearer picture. Stick with me as we explore how predictive analytics works and check out some common examples used today.
What Is Predictive Analytics?
Predictive analytics is the systematic process of using historical data (last month, last year) to establish connections and analyze possible patterns within the data. These relationships and trends, when used on current or future data (tomorrow, next month, next year), can help predict future outcomes.
Artificial intelligence (AI) uses a combination of machine learning applications and statistical techniques to determine the presence and magnitude of changes in one element caused by changes in another within the same data set. After establishing this causality, the AI can apply the same models to new data sets and predict whether there is a change in the second element. For example, you can use historical marketing and sales data to see how significantly specific marketing techniques impact sales.
Why Is Predictive Analytics the Talk of the Town?
In fairness, predictive analytics is nothing new. However, it used to be available only to companies that could afford the resources necessary to carry it out. Only recently has the use of predictive analytics become affordable, easy to use, and therefore popular. It is used increasingly by different organizations in various industries to improve efficiency in everyday operations and achieve that elusive competitive edge.
- Send Marketing Material to Customers That Are Most Likely to Make a Purchase.
Developing a marketing campaign is both a labor-intensive and capital-intensive task. Successful marketing needs to be pertinent and engaging at all levels of your target audience. Therefore, you need to know precisely who is most likely to buy your product and what the commonalities are between such individuals or groups. For example, let us say that your business has a $5,000 budget for a marketing campaign, and you have 100,000 customers. In this case, you clearly cannot give them all a 10% discount. Business intelligence, coupled with predictive analytics, can help forecast the customers that exhibit the most significant probability of buying your product. You can then focus your discounts and coupon books on only those that generate the most revenue.
- Identify Customers Who May Abandon a Service or Product.
Consider, for example, a gym that has implemented a predictive analytics model. Keep in mind that fitness centers usually have a high attrition rate, with many customers dropping their subscriptions reasonably quickly. In this case, let us assume that ‘Jack’ is one such customer that the system identified will not continue the subscription. The basis of judgment is historical data gathered from the gym as well as the data available about Jack. The next time that Jack comes into the gym, the staff will be prepared to offer incentives and discuss the continuation of the relationship between the gym and Jack.
A prominent predictive analysis example comes in the form of Folio 3. It is a company that has developed a solution specifically designed for this problem. Their customer churn prediction system offers advanced customer segmentation, predictive attrition, statistical analysis, and customer retention strategies tailored to potential churners.
- Increase Customer Service Quality Through Precise Planning.
Knowing when customers are most likely to arrive and what they will need is the ideal situation. You can use this information to improve service quality and the overall efficiency of your organization. Through predictive analytics, businesses can forecast demand better by using advanced models and business intelligence. Consider a hotel chain, for example, that wants to know the number of customers that are most likely to visit and stay at specific locations during the Halloween weekend. The hotel can then ensure that they have enough resources and the staff necessary to cater to the flow of customers.
Similarly, a fast-food chain can use predictive analytics to improve drive-thrus and reduce customer wait times. Folio3 successfully developed one such system for its client. Their automated authentication comes from Drive-Thrus. ‘Dashcode,’ the company that wanted the automated system, wished to increase workflow efficiency in Drive-Thrus by breaking down each activity and applying deep learning. In doing so, the system would be able to predict what customers are likely to order and recommend it first to save on order time.
Best Predictive Analytics Examples
No sector is precisely the same and therefore is likely to use predictive analytics in different ways. Here are the top seven industry predictive analytics examples.
1) Sports
One of the most recent additions to predictive analytics in sports is the Microsoft Sports Performance Platform. It is a tool that comes up with data-driven decisions for athletes and teams in almost any aspect of the game, including everything from training schemes for each player to the final composition of the team. The algorithms even help prevent injuries and predict the total recovery time by taking into account factors such as distance traveled and temperature.
A small Danish football club, FC Midtjylland, is an excellent example of predictive analysis. It now analyzes each player twice a month and tailors individual training plans for every player. Analytical models are used to gain insights during the game, make changes to the game plan at halftime, and also suggest new players.
In tennis, IBM introduced a tool named ‘SlamTracker’, which predicts the winner of a match based on a player’s pattern of play, the propensity of forehand use, and willingness to volley. Coupled with computer vision and live game footage, this data can then predict the winner.
2) Retail
Retail is probably one of the most significant examples of predictive analytics. Retailers are always in search of ways to increase customer engagement, loyalty, and retention. One such example is Amazon’s recommendation system. Whenever you make a purchase, the company offers a list of other similar items to the one you just bought. The AI supplies this list based on historical data from other customers who have been buying the same products.
There are many other benefits of predictive analytics in retail, including sales forecasts, market analysis, segmentation, and inventory management. You can also derive revisions to the business models and the best retail locations using predictive analysis. However, it also acts post-sale, reducing returns, getting the customer to come back, and extending warranty sales.
3) Weather
Predictive analytics have become almost indispensable to weather forecasting today. Five-day forecasts are now just as accurate as one-day forecasts from the 1980s. We can now accurately predict the occurrence and movement of large weather systems, including hurricanes, tornadoes, and floods, based on historical data. For example, recently, an extreme polar vortex brought frigid winds down to places like Wisconsin and Minnesota and dropped temperatures to -50 degrees Fahrenheit. The prediction arrived several days in advance, giving people time to prepare. All of this is possible thanks to satellite monitoring of the Earth and complete predictive models that represent how the Earth functions. The movie “Day After Tomorrow” is an excellent example of the use of predictive analytics in assessing the risk of global weather patterns.
4) Health
Predictive analytics in the healthcare sector is focused primarily on how likely it is that an individual will get better or sicker. By analyzing historical data, hospitals can predict which patients are likely to contract a chronic disease and are susceptible to Central-Line Associated Bloodstream (CLAB) infections. This cognitive services process can also help determine the risk of a patient who does not show up for scheduled appointments. Health Catalyst is one such company operating in Salt Lake City since 2008 that specializes in the focus areas mentioned above.
5) Insurance and Risk Assessment
Insurance firms managed to bring losses within risk tolerance levels despite recent financial disasters thanks to predictive analytics. The system ensures that firms set up competitive prices for underwriting, identify fraudulent claims, analyze them, and estimate future losses. Insurance firms can also use predictive analysis to develop marketing campaigns and generate better insights into risk selection and assessment.
6) Financial modeling
Predictive analytics for financial services helps fine-tune the overall business strategy, revenue generation strategy, and resource optimization. Automation of analytics in financial services enables firms to process thousands of models simultaneously and deliver quicker results than with traditional modeling based on manual human interpretation of the data. Human error can severely affect the process of analyzing trends.
Predictive analysis systems can help financial institutions target specific customer segments based on profitability and risk levels. By using historical data, the company can also forecast cash flows and predict demand patterns for specific financial services. Most importantly, the algorithms can alert the firm to customers that deviate from past payment patterns and therefore collect overdue payments much faster.
7) Energy
In the power generation sector, component failures are a real risk and can sometimes cause catastrophic results. The Chornobyl disaster is one such example. By using predictive analytics in power plants, the company can anticipate equipment failures and reduce sudden shutdowns. By predicting when a component will fail, the power plant can use preventive maintenance to address the issue in time and thus reduce maintenance costs and improve the availability of power.
Similarly, utility companies can predict when customers might get a high bill and then alert customers to spikes that occur at certain times of the day. Thereby, the power company can manage the load better and reduce customer complaints.
Predictive Analytics vs. Predictive Modeling
Predictive analytics and modeling serve the same purpose but differ in the methods used. Both systems use historical data and statistical techniques to predict the occurrence of an unknown future event. Predictive modeling examples can be found most commonly in astrology and meteorology.
However, predictive modeling will only give you a probability based on a predetermined modeling framework. On the other hand, predictive analytics uses modeling that is driven by data mining and, therefore, yields more intuitive results. Predictive analytics can predict future events and suggest the best course of action to achieve the best results.
Wrapping Up
Predictive analytics is increasingly becoming a core business function, no matter the size. To succeed and grow their businesses, companies depend on predictive analytics to forecast cash flows, customer engagement, demand, risk, and many more. Predictive analytics and predictive modeling examples are available throughout the business world, improving business insight and, therefore, increasing competency. Now, if you are passionate about growing a business and want to focus on predicting right now, have a look at Folio 3 AI.
Get started with FOLIO3 AI today.
If you’re looking to bring predictive analytics into your business strategy and aim for a 100% success rate, Folio3 AI is here to help. We have you covered with our data-driven insights, where businesses make exceptional decisions and optimize your worth in the market for maximum efficiency and profitability. Our team of experts will be with you every step of the way, ensuring you get the most out of this inventive technology. So, Don’t wait—join the growing number of companies using predictive analytics with Folio3 AI today!
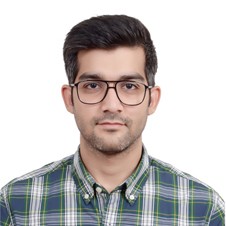
Dawood is a digital marketing pro and AI/ML enthusiast. His blogs on Folio3 AI are a blend of marketing and tech brilliance. Dawood’s knack for making AI engaging for users sets his content apart, offering a unique and insightful take on the dynamic intersection of marketing and cutting-edge technology.