1.0 Introduction
We all know and understand that the food we eat today needs to be treated with different chemicals to increase the food life and sustainability in various conditions. The food we buy from stores contains pesticides that need proper quality control management; otherwise, it can cause harmful results to the customers. The traditional process needs a quality control inspector to manually check the sample of the large production to pass or fail the batch. Still, the sample can inherit some bias, resulting in defective food reaching the customer. Now imagine a world where every bite of food is guaranteed to be safe, fresh, and of the highest quality, where the risk of foodborne illness is minimized, and waste is reduced to a minimum.
This is possible through AI-food detection in supply chain management. AI in recent years came out from the laboratory environment and made serious changes in the world we live in today, from autonomous driving to Amazon warehouse robots, customized suggestions to AR shopping, and the trend continues to the supply chain industry where efficient road selection for logistics, auto box label reading in warehouse started to become the norm. Today we discuss the role of AI in food quality management, its potential benefits, challenges, and the impact it creates by ensuring that the customer receives better quality food every time. I also try to share how to tap into creating solutions for the industry via sharing the current solutions, how they impacted the business involved, and how you can get started!
With all being said, let us explore the benefits of using AI in Food Quality Management.
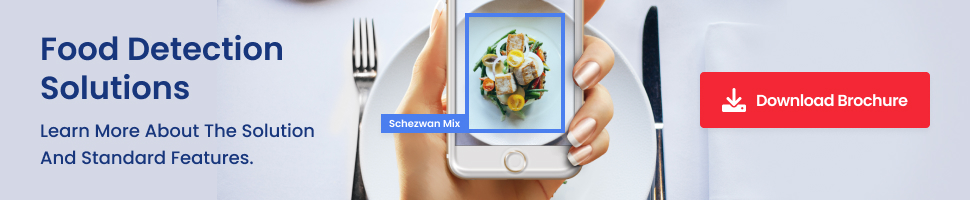
2.0 Benefits
2.1 Foodborne Illness
One of the primary concerns for the food industry is foodborne illness (Dale A. Grinstead, 2015), this not only puts customer health at risk but it also risks the company’s reputation both from the customers and from the government end. A bad reputation opens the company up to legal and strict actions. AI can significantly reduce the risk by identifying contaminated food early in the supply chain, it does so with the usage of computer vision algorithms in detecting visual anomalies in the food. Various algorithms can be tuned for the job, but a custom CNN (convolutional neural network) is typically used to construct the solution from scratch.
A study published in the field demonstrated the power of AI in effectively detecting foodborne pathogens in raw chicken products (Soni, et al., 2022). The study uses the CNN architecture on hyperspectral images of chicken samples. This study demonstrated the practical impact of the AI models in detecting foodborne pathogens, which ultimately helps reduce foodborne illness hence gaining customer trust.
2.2 Quality Control Efficiency
It is necessary to understand that with the ever-increasing population and ever-growing demand, the company’s traditional methods are long due to being updated. The current quality control typically needs manual inspection, which is time-consuming, labor-intensive, and prone to human errors. AI implementation can solve via analyzing the food quality in different parameters (color, texture, size, and shape) and in real-time. This helps the company from manual inspections, speed-up the quality control process, and enables quick decision-making.
A study uses machine learning algorithms to detect food quality defects in apples (Pieczywek, et al., 2018). The author compares machine learning algorithms, including SVM (support vector machine), Decision Trees, and random forests. The paper concluded even simpler AI models are robust enough to identify defects in apples by observing their size, color, and shape.
2.3 Waste Control
Another problem in the food industry is the waste control. The food industry works with a long supply chain, meaning delicate items such as fruits and vegetables lead to food waste occurring in various supply chain stages. AI-based detection systems can be used to identify these defective or spoiled foods early in the supply chain and prevent them from entering the market. This process helps in controlling food waste and also saves
a company from the additional cost of product recalls and returns.
In the paper, researchers use AI-based food detection to detect the freshness of the fish fillets (D., et al., 2021). They utilize hyperspectral imaging and different machine learning algorithms to analyze spectral data in determining the freshness level of fish fillets. This study successfully shows the accuracy of the assessed fish freshness, allowing a business to remove spoiled products and ensure high customer satisfaction.
2.4 Customer Satisfaction
As discussed earlier food industry is highly dependent upon customer feedback and maintaining high-quality management. This management is key to continuously gaining customer satisfaction and establishing a positive brand reputation. AI can help give power to the consumer by digitally enabling them to check the quality of the food and at least the nutrition chart and expiring dates. This enables customers to self-check the food’s health when shopping from the superstore.
A mobile application with a similar AI quality control used in the supply chain can be deployed to the consumer software, enabling them to self-check the fruit quality. This help to gain high customer satisfaction for the company. Another approach to quality
checking can be done through automatic checkout systems, but this is limited to the wide commercial adaption of the automatic checkout system, which has its limitation.
3.0 Challenges
3.1 Data Privacy
Artificial Intelligent Algorithms are primarily dependent upon the data. The accessibility and shareability of this information raise different challenges, including data privacy and regulatory compliance. The food supply chain data composes of sensitive information including products, suppliers, and consumer details; these sensitive data and information must be processed securely to ensure that the data is protected against potential data breaches, cyber threats, and regulatory restrictions. There are different regulations that companies need to follow including General Data Protection Regulation (GDPR)
(Wolford, 2023), Food Safety Modernization Act (FSMA) (US. Food and Drug Administration, 2011), and others.
3.2 Implementation
Although various examples show the potential of the AI solution to the food industry, this implementation does come with a limitation. Current AI models are not robust, meaning the AI model working perfectly in one warehouse may produce errors in the other warehouse of the same company. Additionally, the performance of the AI model is affected by variations in food products, meaning AI models need continuous monitoring and updating to ensure continuous effectiveness in food quality control.
3.3 Human Oversight
Artificial Intelligence enables companies to fasten the quality control process, but it cannot be left on its own. Human oversight is required to verify the accuracy of the AI output (result), evaluate complex situations, and make critical decisions. Artificial intelligence help to automate common tasks but human expertise and judgment are essential for solving exceptional cases. This means effective food quality control and robust solutions require the right balance between AI automation and human expertise.
4.0 Practical Solution
4.1 IBM Food Trust
IBM Food Trust is a promising solution proposed by the repetitive platform enabling transparency in the food industry. The IBM survey concluded that American consumers will pay 36% extra for a responsibly made and transparently sourced product. Over 90% of the consumers surveyed said they would be more loyal to a brand that provides complete transparency (IBM, 2023). IBM Food Trust uses blockchain-based technology to overcome the privacy challenges of the Artificial Intelligent solution. This blockchain approach helps to create an environment where each record is tamper-proof, and transactions between nodes remain transparent.
The application is targeted to solve different problems. Still, one of the major problems it helps is the real-time detection of contaminated products, hence helping reduce the risk of foodborne illness. The other areas IBM Food Trust is helpful are reducing food waste and improving supply chain efficiency. The solution is currently supported by major food companies including the Walmart, Nestle, and Dole, as well as smaller and midsized companies are also started using IBM Food Trust.
Although the IBM Food Trust comes with a promising solution and is supported by the major players in the industry, It is important to mention that the IBM technique has some drawbacks. One of the major drawbacks is the wide-scale testing on the food industry. Blockchain technology is successfully utilized in other industries such as finance and logistics, but the technology is still new to the food industry and needs to be evaluated. Another challenge is the implementation cost of the platform, the big companies can adapt the platform, but the smaller and midsized company adaptability is slow due to the high cost of implementation and business process reengineering.
4.2 IntelligentX Beer
IntelligentX (Matthieu (Harvard Alumni), 2019) beer is a unique brand that uses the power of artificial intelligence to increase engagement with their customer. The company is founded 7 years ago (2016) by HHew Leith and Dr. Rob Mclnerney. The company uses custom algorithms to tailor the flavor and characteristics of the beer according to customer feedback. These characteristics include things like the texture of the bear, bitterness, or sweetness of a bear and others are used to adjust the next batch of beer. One of the direct benefits that the company enjoys is the competitive advantage over other companies in that their beer is tailored to the customer’s need, creating a personal connection with the customer, which increases customer satisfaction and loyalty.
In summary, IntelligentX beer is one of the unique and successful implementations of the food industry that might tell us that artificial intelligence cannot only assess the companies against set quality control but can also be affected in adjusting the quality according to the customer feedback which is something near impossible to manage and produce efficiently with a manual process.
4.3 FarmWise
FarmWise (Heater, 2022) is another AI-based company, that company that is working towards enabling increased productivity and sustainability in farming. The company was founded around the same time as Off IntelligentX (2016) by Sebastien Boyer and Thomas Palomares. The company developed a range of automatic farming machines utilizing computer vision and machine learning algorithms to identify and remove unneeded weeds from fields. There are several challenges associated with the implementation including
the cost of the equipment for the farmers. The other was the connectivity of the data with limited communication in urban areas.
The company successfully utilizes artificial intelligent models to reduce the use of herbicides which is harmful to the environment, it also helps in reducing labor cost and at the same time promote sustainable farming practices. In summary, FarmWise provides an artificially intelligent solution that utilizes robotics, artificial intelligence, and domain experts to increase productivity and sustainability in farming.
5.0 Future Research
Till now, we have learned about the benefits and challenges of AI Food Industry Quality Control, we also explore some related practical implementations, but it is safe to say that although AI-based food quality control in supply chain management where shown promising results there are still improvements and challenges that needed to be solved. In this section, we discuss some promising areas to research to develop mature, robust, and promising solutions for the ever-growing food industry.
5.1 Robust Solution
Current AI quality control solutions are not robust to all conditions and foods. At the start of the conversation, we learned that AI solutions are sensitive to changing conditions and are not equipped to train specifically to one location and then deploy to different locations. There are two areas where the work can be done: developing more robust and mature algorithms that are generalized to food-related parameters and developing a strategy of incorporating data from different locations, and forming a generalized solution. If you are interested in how one develops the second strategy while ensuring data privacy and security try giving it a read to federated learning (Shastri, 2023) and its application.
5.2 Food Quality Accuracy
Many of the approaches shared earlier have one common issue is that we are likely to increase False Positive and False Negative with the condition around AI changes. In simple terms, the algorithm train for a particular set of conditions (which by the way automatically inherited from the data its takes) when exposed to different condition is
likely to increase False Positive (letting go of defective food) and False Negative (marking healthy food defected)*. Comprehensive research is needed to learn what makes the model behave and propose a robust model that limits or decreases False Positives and False Negatives.
*assuming positive refer to food being defective.
5.3 Interpretability of AI Models
With recent advancements in computer vision and deep learning, where on one hand we can increase the robustness and accuracy of the computer vision models, but at the same time we limit our ability to interpret how to model learn features and formulate results. This is important as businesses must understand how a particular model works before adopting a technology that changes their business process. The validation and testing of the algorithm play a key role in ensuring that not only is the model giving good accuracy but it can also be trusted and reliable in the long run.
5.4 Implementation Cost
It is important to understand that AI-based quality control is in its initial phases and is quite expensive to implement. Earlier in the article we discussed how IBM Food Trust although a good solution but the adaptability of the system is limited to the high cost. While this is true when comparing the larger companies to the midlevel and smaller companies, the same is true when comparing the implementation in developing countries vs. underdeveloped countries, there is a huge opportunity to implement existing solutions under limited resources.
6.0 How to get started
Are you feeling it? Yeah, I do too… an interesting opportunity before we drive to the last section of the article. I like to quote something I learn earlier this year while attending the VC Funding session. She said something like this, “I like what young ones are doing with AI, Data Science, Blockchain, and other hot fields but honestly, we prefer old fashion business”. Supply Chain Management is one of the oldest industries, and you can propose a system that can fix small things in the process; it will make a snowballing effect on the industry. Think of Amazon warehouse robots, uber bet on autonomous taxis, and drone delivery; all are related to the existing industry that has been operating for decades and centuries.
Let us now discuss what we can do, starting today to get ourselves prepared. The above solution, benefits, and challenges all can be categorized in a few technical terms. The generic terms that are used to construct the above solution are computer vision (anything that is to do with images and videos), natural language processing (reading text content),
and sound old fashion software engineering. Yeah I know I am a bit oversimplifying things. Still, these are the technical aspects of the articles; keep in mind if you are not a food quality expert you need someone onboard to understand the on-ground challenges of your food quality control, and when you propose a solution, it’s more likely to be the combination of the above three.
6.1 Tools Used
Various tools can be utilized for achieving the above implementation; some of the frameworks are:
Python: Python (Venkat, 2019) is the primary language that is used to conduct exploratory data analysis, automate preprocessing of data, and build pipelines.
Pytorch: Pytorch (Bourke, 2018)Best Suited to develop Deeplearning Architecture for both Computer Vision and Natural Language Processing
Tensorflow & Keras: These (Dhadse, 2021) are well-utilized frameworks for constructing machine learning and deep learning algorithms.
6.2 Technology Used
CNN: Convolutional Neural Network (Prabhu, 2018) is the backbone of computer vision and is typically used to form custom algorithms.
GAN: GANs (Brownlee, 2019) are used to generate augmented datasets that can use to synthesize images, which is useful to generate datasets in case the dataset is limited.
Yolo: You Only Look Once (Kundu, 2023), is a computer vision algorithm used to detect objects in an image or video almost instantly.
FasterRCNN: This (Ananth, 2019) is also a computer vision algorithm best suited for post-processing images and video.
7.0 Conclusion
In conclusion, this article provides an in-depth understanding of the benefits, challenges, and practical implementations of AI-based quality control in the food industry. While the technology has shown promising results in improving food safety and reducing waste, several improvements and challenges still need to be addressed. The article suggests some promising areas for future research, such as developing more robust algorithms, improving food quality accuracy, ensuring the interpretability of AI models, and addressing the implementation cost. Additionally, the article provides some technical terms, tools, and technologies that can be utilized for implementing AI-based quality control solutions. This article provides valuable insights for researchers and industry practitioners looking to leverage AI in the food industry.
As you can see, AI-based quality control has enormous potential in the food industry. However, much work must be done to realize its benefits fully. I encourage you to stay informed about this field’s latest developments and consider how you can contribute to its advancement. Whether you are a researcher, an industry practitioner, or a concerned consumer, there are many ways to get involved. You can attend industry conferences, participate in online forums, and engage with experts in the field. By staying informed and engaged, you can help ensure that AI-based quality control continues to make a positive impact on the safety and quality of our food supply
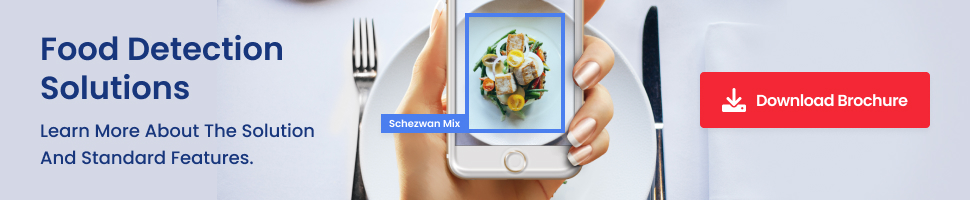
I am Ammar Alam, a data enthusiast, scientist, and Python developer, serving as the Vice-Chairperson of ACM@DSU. With an insatiable curiosity, I uncover insights into complex data, driving positive change. To me, data is not just about numbers or vectors – it is a powerful tool for driving change and making a positive impact. I firmly believe that every piece of data has a story to tell, waiting to be discovered and utilized to solve real-world problems. My vision is a future where data-driven decisions transform industries and society.
—————–
"Implementation of AI-based Food Detection in Supply Chain Management: A Discussion on the Benefits and Challenges of Using AI for food quality control."