Time Series Forecasting with End-to-End MLOPs Service
Summary
Schlumberger is the world’s top oil field service company having average annual revenue of around $30 Billion. Folio3 works with SLB to develop their AI infrastructure and support them with various time-series forecastings having end-to-end MLOPs services on sales data.
About the Customer
Schlumberger Limited is an oilfield services company. As of 2022, it is both the world's largest offshore drilling company and the world's largest offshore drilling contractor by revenue. Schlumberger is incorporated in Willemstad, Curaçao as Schlumberger N.V. and trades on the New York Stock Exchange, Euronext Paris, the London Stock Exchange, and the SIX Swiss Exchange. Its principal executive offices are located in Houston, Texas. In 2022, the Forbes Global 2000 ranked Schlumberger as the 349th largest company in the world.
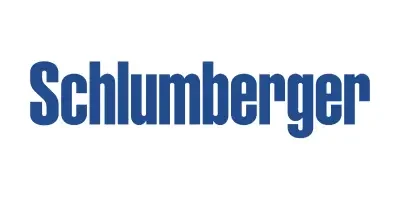
-
Team composition
1 member
-
Client name
Schlumberger
-
Expertise used
Time series analysis & forecasting, Customer classification
-
Duration
5 months
-
Services provided
Vertex AI, MLOPs, GCP Deployment
-
Country
US
-
Industry
Oil Field Services
Solution
The data provided by SLB was trained on both machine learning and deep learning models. For ML, Pycaret library was used while for DL, Darts library was used. Both Pycaret and Darts have multiple ML algorithms to train on the data.
Oil price was also phase shifted by months depending on geographies and business lines. The optimal amount of oil phase shift depends on the max correlation value of revenue and oil price.
Process Automation
Created a training pipeline using GCP Vertex AI that would automate the process of updating big query tables with monthly forecasted results.

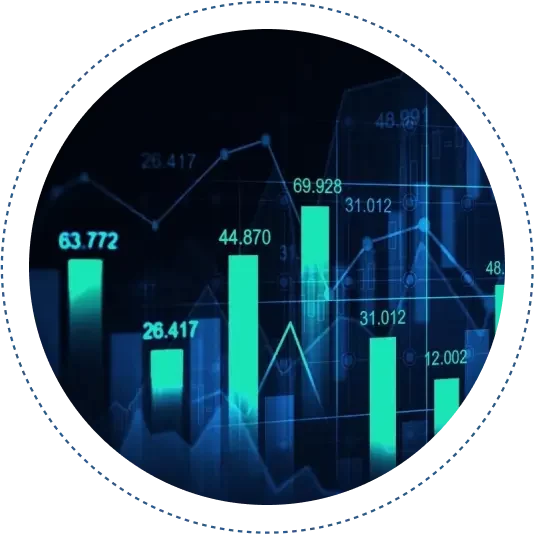

Deployed on Big Query & Google Cloud (GCP)
The solution fetches training data from big query and stores forecasts on the same platform to be used on Google Studio dashboards.
Result
The deployed model helped SLB understand the market trends and demand patterns, helping them optimize pricing, maximizing profit while staying market competitive.