In 2025, precision-driven data strategies are more crucial than ever, requiring generative AI models to process enriched, context-aware inputs for optimal results. Retrieval-augmented generation (RAG) meets this need by combining real-time data retrieval with large language models (LLMs) to produce accurate, context-aware outputs.
Businesses are increasingly adopting RAG to overcome the limitations of traditional AI models, such as outdated information and hallucinations. For example, companies use RAG to improve customer support by ensuring chatbots deliver responses grounded in up-to-date company data, enhance research by pulling the latest market or technical information, and even ease internal processes like document generation and data analysis.
Unlike conventional RAG, which passively pulls data, agentic RAG actively refines queries and adjusts its responses based on real-time feedback, setting the stage for self-governing, self-enhancing AI systems across industries. Read this article for more information about RAG core components and key features.
What is Agentic RAG?
Agentic RAG is an advanced evolution of the Retrieval-Augmented Generation (RAG), designed to make AI more autonomous. While conventional RAG retrieves external information to generate responses, agentic RAG goes further by refining queries, adapting to different contexts, and tackling complex problems. This results in greater intelligence, accuracy, and effectiveness in delivering relevant and insightful responses.
Key Characteristics of Agentic RAG
- Autonomous Query Optimization: A RAG system continuously fine-tunes its queries according to response quality rather than a single retrieval attempt, guaranteeing more relevant and current information.
- Iterative Reasoning & Planning:Β It leverages agentic capabilities to decompose complex issues into organized steps, which improves the coherence and logical progression of generated responses.
- Adaptive Learning & Self-improvement: RAG models can evaluate prior responses, absorb feedback, and adjust their methods to provide more contextually relevant outcomes over time.
- Decision-Making & Task Execution: Unlike static RAG systems, the agents ofΒ RAG can independently identify the most suitable sources, retrieval techniques, and reasoning methods to accomplish tasks efficiently.
Core Components of Agentic RAG
As AI systems progress towards greater autonomy and contextual understanding, Agentic RAG distinguishes itself by combining several advanced elements that improve its dynamic capability to retrieve, reason, and generate responses.
Conventional RAG agents depend on a simple retrieval and generation loop; RAG features intricate mechanisms that facilitate independent decision-making, iterative learning, and contextual adaptation. Below, we examine the key components that drive this state-of-the-art AI framework.
1. Autonomous Agents
Central to RAG is the idea of autonomous agents that function beyond mere passive information retrieval. These intelligent agents evaluate user queries, establish the best search strategies, and enhance retrieval methods in real-time. Traditional agents of RAG merely retrieve documents and produce responses. The autonomous agents in an RAG system are capable of:
- Dynamically Plan and Execute Multi-Step Queries β Rather than fetching information simultaneously, they iteratively adjust queries according to partial results, enhancing response accuracy.
- Prioritize Information Sources β They evaluate various sources, ordering them by credibility, recency, and contextual relevance.
- Adapt to Evolving Inputs β They modify retrieval techniques based on the complexity of the query, guaranteeing that the responses are precise, contextually pertinent, and aligned.
By incorporating agentic capabilities, these AI-powered agents allow RAG to function independently, minimizing human intervention while enhancing response accuracy and contextual depth.
2. Adaptive Retrieval Mechanism
Traditional RAG agents use static retrieval techniques that retrieve documents from a pre-defined knowledge base when queries are made. RAG features an adaptive retrieval system that actively improves and fine-tunes data retrieval according to user interactions and the quality of responses. This enhancement boosts the AI’s capability to secure highly relevant and current information by:
- Iteratively Enhancing Search Queries β The system evaluates initial results and adjusts queries to obtain more accurate information.
- Dynamically Broadening Search Scope β When initial outcomes fall short, the AI expands its search to include external sources, specialized databases, or real-time data streams.
- Assessing Contextual Relevance β Unlike standard retrieval techniques focusing on keyword matching, RAG examines the underlying semantic connections between queries and documents.
This advanced retrieval mechanism ensures that RAG retrieves data and understands, refines, and enhances it, leading to highly relevant and contextually aware responses.
3. Memory and Context Retention
A significant limitation of a conventional agent of RAG is their failure to remember and utilize previous interactions effectively. Agentic RAG overcomes this challenge by introducing memory and context retention, enabling AI models to:
- Uphold Conversational and Task-Specific Memory β Retaining historical interactions facilitates coherent and contextually relevant responses, particularly during multi-turn conversations.
- Detect Patterns in Inquiries β By recognizing recurring subjects, the AI can offer progressive insights, ensuring that responses develop rather than reiterate information.
- Utilize Long-Term Context Awareness β Unlike traditional agents of RAG that consider each query independently, RAG builds on past interactions, enhancing the nuance of its personalized responses.
This component mainly benefits enterprise AI applications, where ongoing conversations and accumulated knowledge enhance decision-making, problem-solving, and user experience.
4. Feedback Loops for Optimization
The true strength of RAG stems from its capability to learn, adapt, and enhance performance through ongoing feedback loops. To traditional RAG agents, which provide fixed responses rooted in pre-existing training data, RAG employs an iterative feedback system that progressively boosts accuracy, efficiency, and contextual understanding.
- Real-Time Performance Evaluation β The system gauges the relevance and correctness of its responses by considering user feedback, engagement metrics, and success rates.
- Automated Refinement of Retrieval & Generation β When an output is identified as inaccurate or lacking, RAG swiftly modifies its retrieval and reasoning processes to enhance the response.
- Self-Learning & Continuous Adaptation β Over time, the AI system sharpens its knowledge base, emphasizing reputable sources while eliminating outdated or irrelevant information.
These feedback loops render RAG exceptionally effective for scenarios requiring critical decision-making, adaptability, and knowledge precision, such as financial analysis, legal research, and autonomous customer support services.
Why Do We Need RAG Agents, and What Do They Solve?
As AI-driven applications increase in complexity, the shortcomings of traditional Retrieval-Augmented Generation (RAG) systems are becoming more apparent. Although agents of RAG, improve language models by fetching pertinent external knowledge, traditional static retrieval models frequently find it challenging to adapt to changing user needs, multi-turn dialogues, and queries that evolve in context.
RAG addresses these issues by incorporating autonomous reasoning, iterative refinement, and adaptive retrieval strategies, ensuring that AI systems stay responsive, intelligent, practical, and highly relevant.
Challenges with Traditional RAG Pipelines
Standard RAG pipelines operate in a relatively fixed manner:
- A query is handled.Β
- Related documents are gathered through keyword or semantic matching.Β
- The AI model creates a response utilizing the retrieved information data.
While effective in many scenarios, this approach has significant limitations when dealing with complex, multi-step, or evolving queries. Some key challenges include:
- Limited Query Adaptation β Conventional RAG models fetch documents using a fixed query that isnβt refined, which frequently results in responses that are either incomplete or incorrect.
- One-Time Information Retrieval β This system fails to evaluate or enhance its retrieval process based on the initial outcomes, resulting in less-than-optimal responses.
- Lack of Context Awareness in Multi-Turn Conversations β AI has difficulty maintaining a consistent understanding throughout several exchanges, often treating each question separately.
- Inability to Handle Complex Reasoning β Traditional RAG systems donβt adaptively strategize their retrieval methods or produce multi-step answers, hindering their effectiveness in analytical and decision-making tasks.
Why Static Retrieval Models Fall Short
Static retrieval models have a fundamental weakness. They rely on a single-pass retrieval process without iterative refinement. This means that:
- Broad or vague queries yield irrelevant results because the system cannot dynamically refine its searches.Β
- Information retrieval falls short in multi-turn interactions, resulting in disjointed responses.Β
- The absence of real-time learning hinders ongoing improvement, as the system does not utilize feedback loops for continuous enhancement enhancement.
For industries requiring accurate, evolving, and personalized responses, such as legal research, medical diagnostics, and enterprise AI solutions, static RAG models simply do not suffice.
How Agentic Models Address These Challenges
To overcome these limitations, Agentic RAG introduces self-improving, context-aware agents that actively refine queries, maintain long-term context, and optimize retrieval processes.
1. Dynamic Query Refinement
Conventional RAG systems that depend on a solitary query-response cycle, RAG persistently enhances queries by evaluating response quality. It achieves this through:
- Iterative Query Optimization β When the first search results fall short, the system adjusts its search settings to obtain more accurate information.
- Context-Aware Query Expansion β The model smartly enhances or restricts the search range depending on the necessary detail level.
- Self-Correcting Mechanisms β If there are inconsistencies or missing information in the retrieved data, RAG modifies its strategy in real time to create more cohesive output responses.
By adapting retrieval strategies dynamically, RAG ensures that responses remain accurate and contextually aligned, even for complex queries requiring multi-step reasoning.
2. Better Handling of Multi-Turn Conversations
Traditional RAG agents frequently struggle with multi-turn conversations since they view each query as separate. RAG maintains memory and context, enabling for:
- Fluent Conversational Flow β The system retains previous conversations, promoting cohesive and logically consistent responses.
- Tailored Follow-Up Questions β RAG enhances its method according to the ongoing discussion instead of reiterating information or overlooking subtleties.
- Customized and Adapting Engagements β The AI modifies its tone, focus, and information retrieval techniques based on the user’s preferences and history of interactions.
This feature is especially beneficial for AI-powered virtual assistants, enterprise chatbots, and knowledge management systems, as it ensures contextual accuracy across several crucial interactions.
3. Context-aware retrieval for Evolving Queries
Static retrieval models struggle with dynamic queries, where users’ intents change over time. RAG addresses this issue by:
- Tracking Query Evolution β The AI detects shifts in user intent and modifies its retrieval priorities accordingly.
- Identifying Relevant Data Sources in Real Time β Rather than depending on static knowledge bases, RAG dynamically queries external databases, APIs, and specialized resources.
- Prioritizing Information Based on Relevance & Recency β The system evaluates, organizes, and modifies retrieval processes to guarantee precise, current, and contextually appropriate responses.
Agentic RAG guarantees that AI models are dependable and adaptable in sectors requiring rapid, data-driven decisionsβlike finance, cybersecurity, and healthcare.
Key Features of a RAG System
Agentic RAG systems signify the next advancement in AI, integrating the strengths of autonomous agents, adaptive retrieval techniques, and ongoing optimization. These systems aim for higher independence, flexibility, and adaptability, overcoming the shortcomings of conventional RAG agents.
Here are the defining features differentiating RAG systems, allowing them to provide more accurate, context-sensitive, personalized results.
1. Autonomous Agents: AI-Driven Agents Dynamically Refining Queries
A standout feature of RAG is its incorporation of autonomous agents. These agents exceed mere query execution and retrieval, significantly contributing to the ongoing enhancement and optimization of the query process. Traditional RAG agents, which depend on a static set of instructions, the autonomous agents in a RAG system possess the ability to:
- Iterative Query Refinement β The agent assesses initial results and adjusts queries to increase relevance and specificity, ensuring that subsequent information retrieval is more targeted and accurate.
- Dynamic Decision Making β Based on initial retrieval, these agents can autonomously refine their search or modify the information-gathering approach, adapting to real-time user needs.
- Contextual Adaptation β As the agent processes each query, it learns from the context and tailors its approach to match evolving user intentions or topic complexity.
By utilizing AI-driven autonomous agents, RAG systems effectively replace static, one-time queries with self-learning, adaptive retrieval cycles that significantly enhance the accuracy and relevance of responses.
2. Adaptive Retrieval Mechanism: Self-Improving Search Strategies
The adaptive retrieval mechanism is another cornerstone of RAG, allowing the system to improve its search strategies constantly based on real-time performance. Self-improving retrieval mechanisms offer several advantages over traditional models:
- Contextual Relevance β Rather than just simple keyword matching, the system assesses more profound semantic relevance, guaranteeing that the content retrieved aligns more closely with the user’s changing query.Β
- Continuous Optimization β The retrieval process continuously improves over time, enhancing accuracy and efficiency. With every query, the system fine-tunes its strategy to prioritize the most pertinent sources, thereby minimizing irrelevant or repetitive information.Β
- Multi-Source Integration β The system can seamlessly gather information from various sources, ensuring thorough coverage of topics and delivering more complete answers to complex queries.
The adaptive retrieval mechanism in RAG leads to a system that is not static but continually evolving and improving based on interactions, ensuring optimal results over time.
3. Memory and Context Retention: Storing and Utilizing Past Interactions
A significant drawback of conventional agents of RAG is their failure to remember the context of prior queries or interactions. RAG integrates memory and context retention features, enabling it to store and use past interactions effectively. This capability is essential for crafting a smooth and tailored experience time:
- Long-Term Context Awareness β The system retains past queries and responses, enabling contextual consistency throughout ongoing interactions.
- Personalized Interactions β RAG leverages stored knowledge to customize responses according to user history, preferences, and previous inquiries, enhancing overall response quality.
- Improved Task Handling β For tasks requiring several steps, the system retains essential data points to ensure a seamless workflow and the systematic gathering of information.
By leveraging memory and context, RAG provides more consistent, relevant, and coherent responses, which is crucial for use cases such as customer support, virtual assistants, and enterprise knowledge management systems.
4. Feedback Loops for Optimization: Iterative Learning to Enhance Accuracy
Integrating feedback loops for optimization allows RAG to continually improve its performance based on the data it receives. Traditional RAG agents generate responses based on predefined models, while RAG systems engage in iterative learning, constantly refining their processes. Key aspects of this feature include:
- Real-Time Learning β The system uses user feedback, response evaluations, and performance metrics to improve its retrieval strategies and response generation.
- Error Correction β If the system generates an inaccurate or incomplete response, it can automatically adjust its approach to refine the outcome in future interactions.
- Self-Improving Mechanisms β Over time, the system adapts its retrieval and generation processes to increase accuracy and decrease response errors, enhancing user performance.
Through feedback loops, RAG becomes a system that learns and evolves, optimizing itself for more accurate, insightful, and relevant interactions.
5. Multi-Agent Collaboration: Coordination Between Specialized Agents
Agentic RAG systems often employ multi-agent collaboration, where multiple agents with specialized knowledge work together to handle complex queries. Each agent within the system focuses on a specific domain, task, or aspect of the information retrieval process, and they collaborate in the following ways:
- Task Division β Different agents specialize in various facets of the query, whether retrieving domain-specific information, handling linguistic nuances, or synthesizing multi-step reasoning.
- Agent Coordination β These agents communicate and share information, ensuring that the best possible outcome is generated based on their collective expertise.
- Optimized Task Execution β By splitting the workload, RAG systems can efficiently handle more complex queries, ensuring accurate, timely, and comprehensive responses.
This collaborative approach allows RAG systems to scale better and process various tasks, especially those requiring expertise across different domains or industries.
6. Self-Correction Mechanisms: Identifying and Fixing Retrieval/Generation Errors
The final key feature of Agentic RAG is its self-correction mechanisms, which allow the system to identify and fix errors in real time. This makes it highly resilient to inaccuracies and errors, especially in high-stakes applications. Key benefits include:
- Error Detection β The system actively monitors the accuracy and quality of its outputs by pinpointing inconsistencies, contradictions, or missing information.
- Automated Error Correction β When an error is detected, RAG proactively modifies its retrieval or generation methods to enhance the accuracy of future responses.
- Continuous Quality Assurance β This self-correcting mechanism ensures high-quality outputs across various applications, including customer support and medical context research.
By implementing self-correction mechanisms, RAG ensures that the system consistently delivers reliable, accurate, and contextually appropriate information with minimal manual intervention.
Benefits of Agentic RAG
Introducing Agentic RAG (Retrieval-Augmented Generation) offers notable benefits, including enhanced accuracy, adaptability, and proficiency in managing complex conversational contexts.
Driven by the advanced features of autonomous agents and adaptive retrieval systems, these advantages could transform numerous applications in AI-driven environment systems.
1. More Accurate and Context-Aware Responses
A significant advantage of Agentic RAG is its capacity to produce highly accurate and context-aware responses. Conventional agents of RAG often depend on static query patterns, which can overlook the subtleties of changing user inputs.
RAG systems consistently enhance their queries and learn from earlier interactions, resulting in responses that better match user intent. This heightened awareness of context leads to improved outcomes in:
- Customized Responses β Interactions are shaped according to the userβs previous inputs, enhancing the overall experience.
- Dynamic Adjustment β The system continually adapts to fresh or unforeseen information, ensuring responses consider the entire conversation or context, not just the immediate question.
- Enhanced Accuracy β By responding to the changing nature of user queries, RAG reduces the presence of irrelevant or unclear information responses.
2. Improved Adaptability in Dynamic Environments
The environment or context constantly shifts in numerous AI applications, necessitating systems to adapt and react to emerging challenges. RAG systems thrive in these dynamic settings, where the type of inquiries, user actions, or external influences can change unpredictably. This adaptability offers essential advantages, including:
- Managing Uncertainty β RAG systems adapt their behavior based on real-time feedback, enabling resilience to abrupt contextual or data changes.
- Self-Improving Skills β RAG models enhance their problem-solving skills through continuous learning and optimization, ensuring relevance amidst environmental shifts.
- Effortless Integration β These systems can connect with diverse data sources and collaborate with other AI solutions, providing versatility for businesses and organizations in dynamic, unpredictable environments and markets.
3. Enhanced Ability to Handle Multi-Turn Conversations
Traditional RAG agents often have difficulty maintaining context throughout extended conversations. Agentic RAG systems excel in these multi-turn interactions, where grasping previous exchanges is essential for delivering pertinent responses. This results in:
- Continuous Context Retention β By remembering and processing data from previous exchanges, RAG ensures a smooth flow in extended conversations.
- Improved Dialogue Management β These systems enhance the handling of intricate dialogues, especially in sectors such as customer support, where conversations change dynamically.
- Personalized Conversations β With the capability to recall past interactions, RAG systems deliver a tailored experience that feels organic and pertinent to the user, improving engagement and satisfaction.
Implementing Agentic RAG: Framework & Tools
A synergy of advanced technologies, frameworks, and tools is essential to implement Agentic RAG systems. These components collaborate to create the infrastructure needed for adaptive, dynamic, and context-aware systems that characterize RAG.
Architectural Overview
The architecture of an Agentic RAG system is designed to support autonomous agents, dynamic retrieval processes, and continuous learning. It typically includes:
- Autonomous Agent Layer β Manages decision-making, enhances queries, and executes tasks.
- Retrieval and Generation Layer β Manages data retrieval, generates responses, and incorporates new knowledge into the system.
- Memory Management Layer β Stores previous interactions and incorporates them into current inquiries.
- Optimization Layer β Employs feedback loops and self-correction methods to enhance the system consistently.
Together, these layers enable RAG systems to be dynamic and self-optimizing, continually improving based on real-time interactions and feedback.
Key Technologies:
To effectively implement an Agentic RAG system, several advanced technologies are critical:
- Large Language Models (LLMs) and Vector Databases β Models like GPT-4 and BERT drive RAG systems’ generation and comprehension features. They create and interpret human-like text, while vector databases hold semantic data representations, enabling quick access and enhancing response relevance.
- Reinforcement Learning for Optimization β By employing reinforcement learning (RL), the system gathers feedback to improve its performance over time. This technique is particularly beneficial for honing query generation and data retrieval methods, ensuring that RAG consistently enhances efficiency.
- Multi-Agent Systems (MAS) in AI β Multi-agent systems facilitate cooperation among specialized agents, leading to more intricate and detailed interactions. Various agents can concentrate on distinct tasks or fields, collaborating effectively to deliver optimal outcomes.
Use Cases of Agentic RAG
The unique advantages of RAG make it an ideal solution for a wide range of industries and applications, from customer support to data analysis. Below are some of the key use cases where RAG can make a substantial impact:
- Customer Support and Virtual Assistants β RAG significantly improves customer support systems by managing multi-turn conversations, maintaining context, and responding to user demands in real time. It delivers accurate, context-sensitive replies that feel natural and tailored to each user.
- Healthcare and Medical Research β RAG assists healthcare professionals by dynamically sourcing and synthesizing pertinent information and adapting to changing research requirements or evolving patient conditions.
- Enterprise Knowledge Management β Within companies, RAG systems can consistently enhance access to internal knowledge bases, ensuring employees receive timely and accurate information when needed.
- Personalized E-commerce β RAG refines product recommendations for online retail platforms and fosters customized shopping experiences based on past interactions and shifting customer preferences.
- Legal and Compliance β In the legal field, RAG aids lawyers and compliance experts in obtaining relevant legal documents, case law, or compliance guidelines, ensuring that contextual relevance is preserved throughout the retrieval process.
The potential applications of RAG are vast and growing, offering innovative, dynamic solutions to businesses and industries across the board.
Conclusion
Agentic RAG represents a significant advancement in AI, integrating autonomous agents, adaptive retrieval, and continuous learning to address the shortcomings of traditional agents of RAG. to static retrieval models, it actively refines queries, maintains context throughout multiple interactions, and enhances accuracy via feedback mechanisms.
By utilizing LLMs, vector databases, and reinforcement learning, Agentic RAG fosters the development of more context-aware, adaptable, and intelligent AI systems. Its uses extend across various sectors, including customer support, healthcare, and enterprise AI, positioning it as a transformative solution for businesses aiming for more innovative, more responsive AI-driven initiatives.
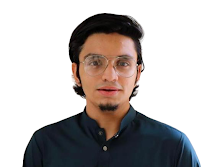
Areeb is a versatile machine learning engineer with a focus on computer vision and auto-generative models. He excels in custom model training, crafting innovative solutions to meet specific client needs. Known for his technical brilliance and forward-thinking approach, Areeb constantly pushes the boundaries of AI by incorporating cutting-edge research into practical applications, making him a respected developer in folio3.