The drug discovery process is a complex and arduous journey, often spanning over a decade and requiring substantial financial investment. On average, bringing a new drug to market can take 10 to 15 years and cost approximately $2.5 billion.
Despite these investments, the success rate remains low, with about 90% of drug candidates failing during clinical trials, primarily due to issues related to efficacy and unexpected toxicity. These challenges underscore the need for more efficient and effective methodologies in drug development.Â
Generative Artificial Intelligence (AI) has emerged as a transformative tool in addressing the inherent challenges of drug discovery. With intensive datasets, generative AI can design novel molecular structures, predict their interactions with biological targets, and optimize potential drug candidates with unprecedented speed and accuracy.
This capability not only accelerates the initial phases of drug development but also enhances the likelihood of clinical success by identifying promising compounds early in the process. As pharmaceutical companies strive to reduce costs and improve outcomes, the integration of generative AI into drug discovery has become increasingly vital.Â
To delve deeper into the practical applications of generative AI in drug discovery, including its role in molecule generation, biomarker identification, and drug repurposing. Must, read this blog.
Applications of Generative AI in Drug Discovery
The role of Generative AI, especially in drug discovery, might be esoteric. Still, it has really changed the pharmaceutical landscape by accelerating drug discovery processes that traditionally spanned years and cost billions. Generative models like VAEs, GANs, and transformers use deep learning to generate new biological and chemical structures, improving efficiency and revealing new therapeutic possibilities.
Below are five key applications of generative AI in drug discovery.
1. De Novo Molecule Generation
One of the most prominent uses of generative AI is in de novo molecule generation, where new drug-like molecules are created from scratch. Unlike traditional screening methods that rely on pre-existing compound libraries, generative models can design entirely novel molecular structures with desired biological properties.
VAEs and GANs are particularly useful in this context, as they can learn the latent space of chemical structures and generate new molecules optimized for potency, solubility, and selectivity.
These AI-designed molecules can then be synthesized and tested, dramatically reducing the time and cost involved in early-stage drug discovery.
2. Protein Structure Prediction
Understanding a protein’s 3D structure is indispensable for drug targeting. However, Generative AI models have made significant strides in this domain. Tools like AlphaFold and RoseTTAFold, which use deep learning techniques including transformers and attention mechanisms, have reshaped protein structure prediction by achieving near-experimental accuracy.
These models generate atomic-level predictions of protein folding based on amino acid sequences, enabling researchers to identify binding sites, predict interactions with potential drugs, and design more precise therapeutics. This leap in accuracy and accessibility opens doors for targeting previously undruggable proteins.
3. Drug Repurposing
Generative AI also facilitates drug repurposing, the process of identifying new therapeutic uses for existing drugs. Generative language models (such as GPT-based systems) can suggest new drug-disease associations by analyzing vast biomedical literature, gene expression profiles, and clinical data. These models can simulate how existing compounds might interact with alternative targets or pathways, offering faster routes to clinical application.
This is especially valuable during health emergencies like pandemics. Moreover, integrating generative AI with knowledge graphs enhances the ability to uncover non-obvious links across complex biological systems.
4. Predicting ADMET Properties
A major hurdle in drug development is predicting how a drug will behave in the body, summarized by ADMET (Absorption, Distribution, Metabolism, Excretion, and Toxicity) properties. Generative AI models are now being trained to forecast these pharmacokinetic and toxicological profiles early in the discovery pipeline.
Using molecular descriptors and learned chemical representations, models like graph neural networks (GNNs) and VAEs can predict how a molecule might be absorbed, where it might distribute, how it is metabolized, its excretion pathways, and potential toxicities. These insights help in eliminating unsuitable candidates early, thereby reducing late-stage failures.
5. Synthesis Planning
Once a promising compound is identified, the next challenge is determining how to synthesize it. Generative AI is being used for retrosynthetic analysis, where the target molecule is broken down into simpler precursors. AI models can predict feasible reaction pathways using large-scale reaction databases and rule-based logic combined with machine learning.
Transformer-based models trained on chemical reaction data (like the USPTO dataset) are now capable of suggesting step-by-step synthesis routes that are both efficient and cost-effective. This assists chemists in rapidly scaling up the production of drug candidates for further testing.
FAQ
How Does AI Help in Drug Discovery?
AI speeds up drug discovery by predicting molecular interactions and uncovering new therapeutic targets.
Is Generative AI in Drug Discovery Overhyped?
Generative AI shows promise but still requires refinement for consistent, reliable results in drug discovery.
Final words
The importance of generative AI in drug discovery cannot be overstated. With the pharma industry facing high R&D costs, long timelines, and a lot of failures, this technology offers a much-needed shift. It helps design new molecules quickly, predict protein structures accurately, repurpose existing drugs, and even forecast properties like ADMET.
Plus, it speeds up drug development by simulating biological interactions, making candidate selection smarter, and cutting down inefficiency and toxicity. Integrating it is the key to making drug discovery faster, smarter, and more cost-effective.
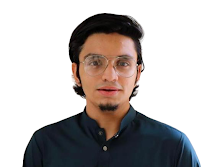
Areeb is a versatile machine learning engineer with a focus on computer vision and auto-generative models. He excels in custom model training, crafting innovative solutions to meet specific client needs. Known for his technical brilliance and forward-thinking approach, Areeb constantly pushes the boundaries of AI by incorporating cutting-edge research into practical applications, making him a respected developer in folio3.