The sports industry is now a major hub for AI, where detailed game analytics are highly valued. In baseball, AI-powered video analysis has emerged as a lucrative solution, turning hours of captured footage into nuanced insights that reveal the hidden narratives behind every pitch, hit, and defensive maneuver. These AI-powered sport video analysis don’t just record the game—they break it down.
Data such as pitch velocity, spin rate, launch angle, player positioning, and reaction time are captured to provide a clearer understanding of performance and strategy.
For example, in the USA, Major League Baseball (MLB) teams rely on Statcast to track the precise movement of the ball and players. This allows coaches to analyse how fast a ball left the bat, how well a fielder covered ground, or where a pitcher is most likely to succeed. These insights are no longer optional—they’re a competitive edge.
Must read to explore baseball data analytics has become such a critical part of sports and how it’s changing the way the game is played, coached, and experienced.
First, we’ve compared traditional statistics with modern AI-powered analytics to show how sports Analysis helps coaches to be ahead of the game.
Traditional Baseball Statistics vs. Modern Analytics
This table highlights the shift from traditional baseball stats to AI-powered analytics. While traditional stats were the foundation, AI baseball sports video analysis solutions offer deeper analysis into player performance and strategy. These data-driven methods help teams make cogent decisions and also can pinpoint where a team is lacking , with proper demo graphics , giving them a clear advantage over primitive methods.
Aspect | Traditional Baseball Statistics | Modern Analytics (AI-Powered) |
Focus | Basic outcomes (hits, runs, RBIs, wins) | In-depth performance metrics and predictive insights |
Common Metrics | Batting Average, ERA, Home Runs, RBIs | Exit Velocity, Spin Rate, Launch Angle, WAR, xBA, xERA |
Data Collection | Manual recording and observation | Automated tracking via AI, sensors, and video analysis |
Decision-Making Use | Based on experience and traditional stats | Data-driven decisions using machine learning models |
Player Evaluation | Relies on surface-level stats | Evaluates performance potential and consistency over time |
Technology Involvement | Minimal | High – involves AI, computer vision, and real-time data feeds |
Strategic Impact | Limited to basic adjustments | Enables dynamic game-time strategy shifts and player matchups |
Examples | Batting .300 is considered excellent | A hitter’s high xwOBA may indicate better future performance |
Key Metrics and Concepts in Baseball Analytics
These metrics and concepts are important for various people in baseball. Coaches and managers use them to improve strategies and player performance. Scouts use analytics to find and evaluate new talent. General Managers and team executives rely on these data to make decisions on player trades, contracts, and team building. Players like pitchers and hitters use the data to improve their skills, while analysts help interpret the data to guide decisions and enhance team success.
1- On-base Plus Slugging (OPS)
OPS combines a player’s ability to get on base (On-base Percentage, OBP) and their power-hitting ability (Slugging Percentage, SLG). This statistic gives a comprehensive view of a batter’s overall offensive performance. The formula for OPS is:
2- OPS = OBP + SLG
Higher OPS values indicate a more productive hitter, as it accounts for both reaching base and hitting for power. Launch Angle and Exit Velocity are two metrics that directly influence a batter’s slugging percentage, as they affect the quality and distance of hits. A batter with a high OPS is likely hitting with both power and consistency, which is a reflection of their overall offensive value.
3- Wins Above Replacement (WAR)
WAR quantifies a player’s total contributions to their team, both offensively and defensively, and compares that value to a “replacement-level” player—someone who can be easily substituted into the roster without a significant drop in performance. A player with a WAR of 1.0 or higher is generally considered above average, while those with WARs above 5.0 are elite players.
WAR is influenced by multiple stats, including OPS (for offensive contributions) and FIP (for pitchers). For example, a player with a high BABIP (indicating they get a lot of hits on balls in play) may have a higher offensive WAR, while a pitcher with a low FIP might have a higher pitching WAR.
4- Fielding Independent Pitching (FIP)
FIP is a statistic designed to assess a pitcher’s performance independent of the defense behind them. It focuses on the three true outcomes a pitcher controls: strikeouts, walks, and home runs. The formula for FIP is:
FIP = (13 * HR + 3 * BB – 2 * K) / IP + constant
This metric is valuable because it isolates a pitcher’s skill level from fielding errors or defensive plays that could affect their performance. A pitcher with a low FIP is often more effective in generating strikeouts and preventing home runs. A pitcher with a strong FIP will contribute positively to their team’s WAR, making them a more valuable player overall.
5- Batting Average on Balls in Play (BABIP)
BABIP measures how often a batter reaches base via a hit on balls they put into play (excluding home runs). It reflects the luck or skill involved in batting, as it is affected by factors such as the defense, the quality of contact, and weather conditions. The formula for BABIP is:
BABIP = (H – HR) / (AB – K – HR + SF)
An unusually high or low BABIP can indicate a player’s performance may be unsustainable in the short term. Players who consistently hit for high BABIP are often those who hit the ball with authority and have a favorable launch angle. For example, batters who hit line drives with high exit velocity tend to have higher BABIP rates, suggesting they’re more likely to get base hits.
6- Launch Angle and Exit Velocity
Launch Angle is the vertical angle at which a ball leaves a bat, while Exit Velocity measures the speed of the ball as it leaves the bat. Both are key to understanding a batter’s offensive potential, as they directly influence the likelihood of a ball leaving the park or resulting in extra-base hits. High exit velocity and an optimal launch angle (typically between 10 and 30 degrees) increase the chances of hitting for power.
These two metrics are closely connected to OPS.
Batters who consistently achieve high exit velocity and optimal launch angle are more likely to produce extra-base hits, thus improving their slugging percentage, which contributes to a higher OPS. Furthermore, batters with better launch angles and exit velocities tend to have higher BABIP, as they make better contact and hit the ball harder, increasing the chances of getting on base.
Technologies Powering Baseball Analytics
AI-Powered solutions like deep learning, computer vision, and real-time data collection add value to baseball by providing insights that improve performance. Deep learning uncovers patterns in data, computer vision analyzes player movements and pitches, while real-time data collection tracks metrics like pitch speed and exit velocity. These technologies facilitate teams in deriving actionable insights, fostering superior decision-making, and securing a formidable edge in the competitive landscape.
AI-Based Video Tagging for Faster Insights
AI automates the process of tagging video clips, identifying key events like pitches, hits, and defensive plays in real time. This saves analysts countless hours and helps coaches quickly review specific moments. The system can highlight patterns and player behaviors that would otherwise go unnoticed in manual reviews.
1- Statcast System (Tracking Player Movements and Ball Trajectory)
Statcast, used by Major League Baseball (MLB), combines radar and high-speed cameras to measure every movement on the field. It tracks pitch velocity, exit speed of the ball, player speed, and positioning—turning each play into measurable data. This gives teams detailed insights into player efficiency and ball dynamics.
2- Radar, Cameras, and Motion Sensors
Advanced tracking tools like radar systems, HD cameras, and wearable sensors capture everything from pitch spin to a player’s running form. These tools work together to build a full picture of game dynamics, allowing for more accurate analysis of mechanics, fatigue, and field coverage.
3- Machine Learning and Predictive Modeling in Baseball
Machine learning algorithms analyse large volumes of data to spot trends, predict outcomes, and optimise game strategies. Teams use predictive models to assess player performance, injury risk, and game scenarios. This data-backed foresight supports smarter decisions in scouting, training, and in-game tactics.
Applications of Data Analytics in Baseball
1. Player Scouting and Recruitment
Data analytics helps teams evaluate player potential beyond traditional stats like batting average or home runs. Advanced metrics such as on-base plus slugging (OPS), Wins Above Replacement (WAR), and exit velocity allow for more accurate assessments.
Example:
The Oakland Athletics’ Moneyball approach, made famous by the book and movie, used analytics to identify undervalued players who had high on-base percentages. This helped them build a competitive team on a limited budget.
2. In-game Strategy and Decision Making
Teams use real-time data to guide tactical decisions such as pitch selection, defensive shifts, or when to use pinch-hitters. These decisions are based on historical performance data, player matchups, and situational probabilities.
Example:
The Houston Astros and Los Angeles Dodgers have used analytics to position their defenders perfectly for each batter’s tendencies, often shifting infielders dramatically to increase the chances of making outs.
3. Injury Prevention and Load Management
Wearable sensors and data tracking tools monitor players’ physical exertion, biomechanics, and fatigue. This helps coaches avoid overuse injuries and manage player workloads across a long season.
Example:
Teams like the Los Angeles Dodgers use motion capture systems and biomechanical assessments to predict when a pitcher may be at risk of elbow or shoulder injuries, adjusting rest schedules accordingly.
4. Fan Engagement and Broadcast Enhancements
Data-driven insights enhance the viewing experience with visual graphics, real-time stats, and predictive models shown during live broadcasts. Fans also engage more deeply with fantasy leagues and social media content based on player stats.
Example:
Statcast, used by MLB broadcasts, provides data like pitch velocity, spin rate, and catch probability, giving fans a deeper understanding of the game and players’ performance.
Case Studies: Analytics Success Stories
1. Oakland Athletics – The Birth of Moneyball
In the early 2000s, the Oakland Athletics, under General Manager Billy Beane, revolutionized baseball by adopting a data-driven approach to player evaluation. Facing budget constraints, they focused on undervalued metrics like on-base percentage (OBP) to assemble a competitive team. This strategy led to a remarkable 20-game winning streak in 2002 and a playoff appearance, despite having one of the league’s lowest payrolls. The success of this approach was chronicled in Michael Lewis’s book “Moneyball,” highlighting the impact of analytics in baseball.
2. Houston Astros – From Rebuilding to World Series Champions
The Houston Astros underwent a significant transformation by embracing analytics. After a series of losing seasons, they hired Jeff Luhnow as General Manager in 2011, who prioritized data-driven decision-making. By leveraging advanced analytics for player development and in-game strategies, the Astros improved their performance dramatically, culminating in winning the World Series in 2017. This turnaround showcased the power of analytics in building a championship team.
3. Los Angeles Dodgers – Pioneering Injury Prevention with Analytics
The Los Angeles Dodgers have been at the forefront of using analytics to prevent player injuries. By partnering with companies like Kitman Labs, they implemented systems to monitor players’ physical data, aiming to predict and prevent injuries before they occur. This proactive approach has been instrumental in maintaining player health and optimizing performance throughout the season.
Conclusion
The cumulative value of AI-powered video analysis lies in its ability to turn raw footage into actionable insights—instantly, accurately, and at scale. In the context of baseball and beyond, this AI-powered sport video analysis with baseball data analytics changing the perception of decisions. From refining player performance and optimizing team strategy to enhancing fan engagement and reducing injury risks, video analytics provides a competitive edge that manual observation simply cannot match.
As industries increasingly integrate AI-driven tools, the real-time decision-making power of video analysis enables faster responses, deeper pattern recognition, and continuous improvement. Whether it’s sports, healthcare, security, or manufacturing, the impact is universal—transforming processes, increasing precision, and unlocking new levels of efficiency.
Looking forward, the potential of AI-powered video analytics continues to expand. With advancements in computer vision, edge computing, and machine learning, we are only beginning to scratch the surface of what’s possible. Industries that embrace this evolution today are positioning themselves not just to keep up, but to lead and win in tomorrow’s data-driven world.
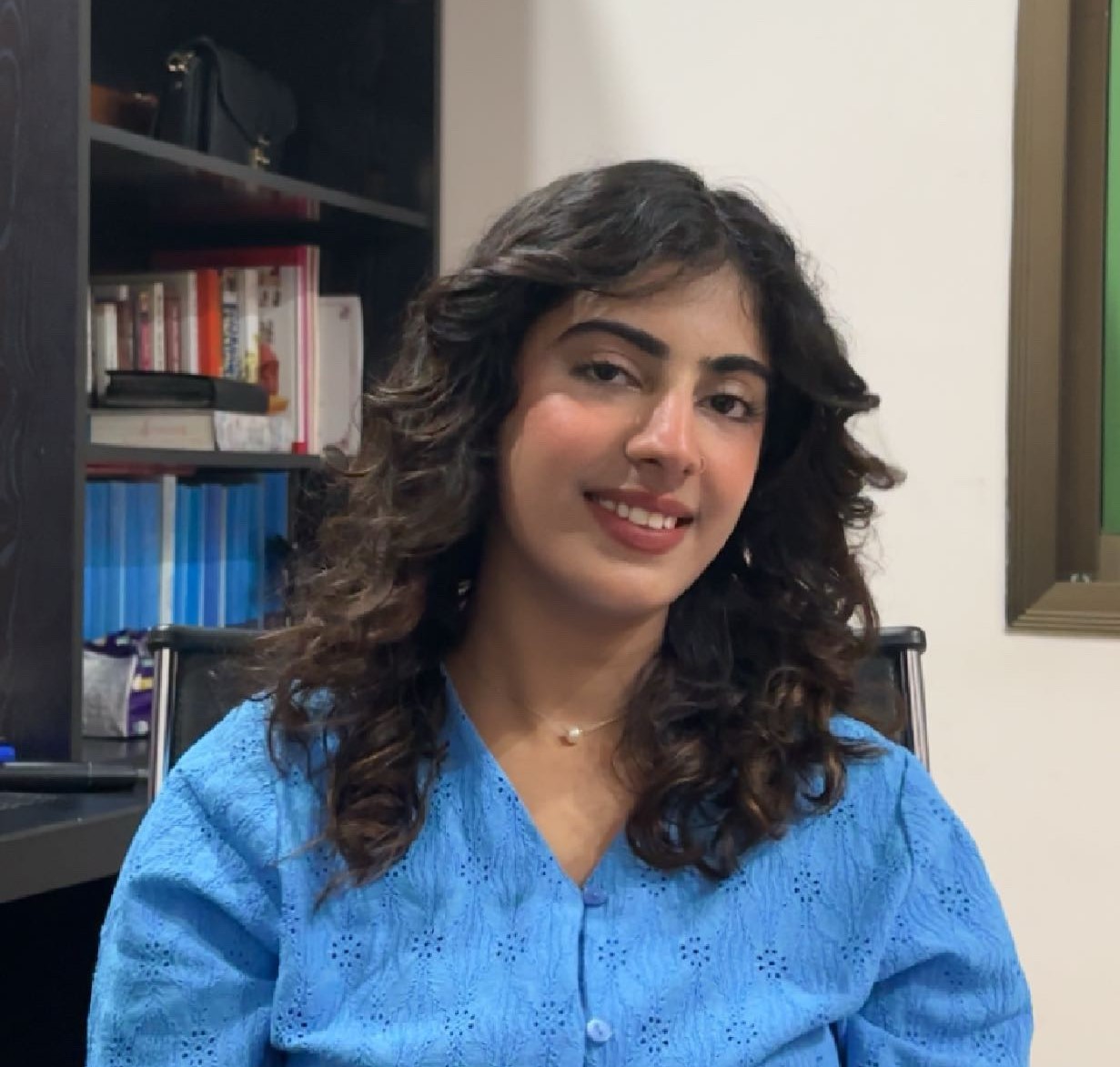
Manahil Samuel holds a Bachelor’s in Computer Science and has worked on artificial intelligence and computer vision She skillfully combines her technical expertise with digital marketing strategies, utilizing AI-driven insights for precise and impactful content. Her work embodies a distinctive fusion of technology and storytelling, exemplifying her keen grasp of contemporary AI market standards.