AI Agent Orchestration focuses on overseeing and synchronizing AI agents to collaboratively achieve a unified goal. It is increasingly necessary as AI-driven environments become more complex.
It solves the challenge of fragmented automation by creating a unified framework where specialized agents can cooperate effectively. Rather than relying on a single monolithic AI system, companies can deploy several focused agents, each tailored for a specific task, and use orchestration to make them work in synergy.
For example, consider a modern e-commerce platform. One AI agent might specialize in product recommendations, analyzing user data to suggest relevant items. Another agent could be dedicated to customer service, handling queries through a chatbot interface. A third agent might be managing inventory and logistics, ensuring timely order fulfillment. Without orchestration, these agents would operate independently, leading to communication gaps and inefficient workflows.
However, with AI Agent Orchestration in place, these agents collaborate, and the recommendation system informs the chatbot of relevant product suggestions, which then communicates purchase data to the logistics agent for the fulfillment, all happening autonomously and in real-time.
This improves efficiency, scalability, and flexibility within AI ecosystems. As Raza Habib, CEO of Humanloop, aptly puts it, “The future of AI systems is not just building smarter individual agents, but in how effectively we can orchestrate them to solve real-world problems.”
To understand this topic in even more depth, including the types of orchestration techniques and real-world implementations, continue reading as we explore the practical side of how businesses are adopting and benefiting from AI Agent Orchestration.
Types of AI Agent Orchestration
The growing importance of AI Agent Orchestration is fueled by the need for operational efficiency at scale. Businesses can now manage large-scale AI deployments without sacrificing agility or control.
Moreover, it allows for specialization, where companies can select or build the best AI agent for each task and plug them into the orchestrated system. However, there are different types of AI agent orchestration.
Centralized Orchestration
Centralized orchestration uses a single controller to manage all AI agents from one point. This model acts like a command center and assigns tasks to agents based on a shared goal. It’s easy to implement and works well in smaller or integrated environments, such as customer service systems with chatbots, recommendation engines, and payment systems.
However, in more complex setups, it can create bottlenecks since all agents rely on one controller.
Decentralized Orchestration
Decentralized orchestration eliminates the need for a central controller, allowing agents to operate independently and communicate directly with each other. Each agent makes decisions based on its local environment and interactions with peers.
This model is well-suited for large, distributed systems like fleets of autonomous vehicles or IoT networks, where quick responses to local changes are crucial. While it offers flexibility and resilience, it can be harder to design and manage due to the complexity of inter-agent communication.
Hybrid Orchestration
Hybrid orchestration combines both centralized and decentralized models. Some functions are managed by a central orchestrator, while others are handled autonomously by individual agents. For instance, in a smart factory, a central system oversees production planning, while robotic arms adjust based on real-time sensor data.
This model strikes a balance between centralized control and local adaptability, making it ideal for dynamic environments that need both structure and flexibility.
Hierarchical Orchestration
Hierarchical orchestration organizes AI agents into layers or tiers, with higher-level agents managing groups of agents below them in a tree-like structure. Similar to large organizations, where senior managers oversee mid-level managers who guide frontline staff, this model is common in complex systems like multinational logistics networks or AI ecosystems across business units.
It helps distribute workloads while maintaining overall control at higher levels.
Swarm-Based Orchestration
Swarm-based orchestration is inspired by natural systems, such as flocks of birds or ant colonies. Individual agents follow simple rules and interact locally, but together, they create a coordinated and intelligent outcome. There is no central controller, yet the system remains organized and purposeful.
This model is highly scalable and resilient, making it ideal for scenarios such as drone swarms, automated warehouse robots, or search-and-rescue missions, where flexibility and robustness are essential.
Event-Driven Orchestration
Event-driven orchestration activates agents based on specific events or conditions. Rather than constantly receiving instructions, agents stay idle until an event, Such as a system anomaly or user action, triggers them. Once activated, agents collaborate to respond autonomously.
This model is efficient and responsive, making it ideal for applications like cybersecurity, where immediate action is needed in response to threats, or monitoring systems that act only when unusual patterns are detected.
Key Components of AI Agent Orchestration
For AI Agent Orchestration to function incisive, several crucial components are required. These ensure that agents collaborate smoothly, rather than operating in isolation.
Agent Communication Frameworks
First, you need a way for all these AI agents to talk to each other, and that’s what agent communication frameworks do. This is like the shared language or communication highway that allows agents to exchange information and instructions.
Even if it’s passing customer data from one bot to another or sharing status updates between warehouse robots, without this communication layer, agents wouldn’t be able to collaborate. These frameworks can be designed using protocols like APIs, message brokers, or custom-built communication systems that keep everything connected in real time.
Task Allocation and Coordination
Once the agents can communicate, the next challenge is deciding who does what. That’s where task allocation and coordination step in. This component ensures that tasks are assigned to the right agents based on their capabilities and availability.
Think of it like a project manager handing out tasks to team members based on their skills and current workload.
For example, if a logistics agent is busy, the orchestrator might redirect a delivery task to another available agent. Good coordination prevents bottlenecks and helps the system run smoothly.
Decision-Making Models
Another key piece is how agents (or the orchestrator) make decisions. These decision-making models can be rule-based, AI-driven, or a combination of both. Some systems rely on simple if-then rules, while others use machine learning models to predict outcomes and make smarter choices.
For instance, should the system escalate a customer complaint to a human agent or let the chatbot continue handling it? Decision models help the agents make informed choices depending on the situation, data, and goals.
Scalability and Adaptability
AI agent orchestration also needs to be scalable and adaptable. As systems grow, maybe you go from managing 10 agents to 1,000—the orchestration framework must handle that increase without falling apart. It should also adapt to new agents or tasks easily.
For example, you might start with basic customer service bots but later want to add AI agents for marketing automation or supply chain management. A good orchestration system should plug these new agents in smoothly without requiring a total system overhaul.
Security and Governance
Lastly, security and governance are crucial. With multiple agents exchanging sensitive data and making autonomous decisions, you need strict rules around who can do what and how data is protected. This includes setting access controls, monitoring agent behavior, and ensuring compliance with regulations like GDPR or industry standards.
Proper governance also helps avoid situations where agents might conflict or make unauthorized decisions that could harm the system or organization.
Steps to Implement AI Agent Orchestration
AI Agent Orchestration is quickly becoming the backbone of modern AI-powered operations. Implementing it in your business environment requires taking some pragmatic steps.
Step 1: Define Objectives and Use Cases
The first step is to define clear objectives and identify use cases where AI Agent Orchestration adds value. Align the strategy with business goals, such as improving customer experience or automating processes. This helps determine the types of agents, scale, success metrics, and the right technologies for implementation.
Step 2: Design the AI Agent Framework
Once objectives are set, the next step is to design the AI agent framework. Choose an orchestration model (centralized, decentralized, hybrid, etc.) based on your system’s complexity and scalability.
Define each agent’s role, how they interact, and how tasks flow. Consider system constraints, data sources, integration with existing infrastructure, and agent interoperability.
Step 3: Develop AI Agents and Capabilities
With the framework in place, the next step is to develop AI agents with specialized capabilities, such as NLP for chatbots, predictive analytics for forecasting, or computer vision for inspection. Use pre-trained models, APIs, or custom solutions as needed, and integrate them with real-time data pipelines to ensure effective task execution.
Step 4: Establish Communication and Coordination Mechanisms
After developing the agents, set up the communication framework using APIs, message brokers (e.g., RabbitMQ, Kafka), or protocols like FIPA-ACL. Define how agents will share data, negotiate, and escalate tasks, ensuring synchronization and conflict avoidance through coordination mechanisms like task allocation algorithms.
Step 5: Deploy AI Agents in a Scalable Environment
Deployment brings your agents to life in the real world. Choose an environment that supports scalability, such as cloud platforms (AWS, Google Cloud, Azure) or edge computing for latency-sensitive tasks. Use containerization tools like Docker and orchestration systems like Kubernetes to ensure scalability and high availability.
This phase also involves integrating agents with external systems, such as CRM, ERP, or IoT networks, based on the use case.
Step 6: Monitor and Optimize Performance
Once live, continuous monitoring is crucial. Use dashboards and tools like Prometheus, Grafana, or ELK stack to track performance, response times, success rates, and errors. AI agent orchestration should evolve, with monitoring insights guiding regular optimization, such as retraining models or refining coordination. The goal is to maintain efficiency, resilience, and alignment with changing business needs.
Step 7: Implement Security and Compliance Measures
Lastly, security and compliance are crucial, especially post-deployment. Implement strong access controls (RBAC), and encryption for data in transit and at rest, and conduct regular security audits. Ensure compliance with regulations like GDPR, HIPAA, or industry-specific standards.
Establish governance policies to define agent interactions, decision escalations, and handling of sensitive information, ensuring long-term system integrity and trust.
Best Practices for AI Agent Orchestration
After the implementation of AI Agent orchestration in your work environment. It requires best practices that prioritize system efficiency, scalability, and security. Here are the main factors to consider
- Design for Scalability: Build AI systems with scalability in mind using cloud platforms and containerization (e.g., Docker, Kubernetes) to handle varying workloads without sacrificing performance.
- Ensure Interoperability: Use standardized communication protocols and APIs to enable AI agents to work together smoothly, regardless of the technology they use.
- Optimize for Real-Time Decision-Making: Implement real-time data processing and low-latency communication to help AI agents respond quickly to changing conditions.
- Prioritize Security and Privacy: Use encryption, secure authentication, and regular security audits to protect sensitive data and comply with regulations like GDPR or HIPAA.
- Continuously Train AI Agents: Set up continuous learning pipelines to keep AI agents updated with new data, ensuring they stay effective and aligned with business needs.
Final Words
AI Agent Orchestration is rapidly becoming a cornerstone for building intelligent, scalable, and efficient AI-driven ecosystems. It manages autonomous vehicles, optimizes supply chains, and enhances customer service platforms, enabling organizations to unlock higher levels of automation, adaptability, and operational excellence.
However, success depends not just on deploying individual agents, but on how effectively they are coordinated to work as a unified system.
By following best practices like designing for scalability, ensuring interoperability, prioritizing security, and fostering continuous learning, businesses can future-proof their AI systems. As industries move toward more complex and distributed AI environments, mastering orchestration will become a crucial competitive advantage.
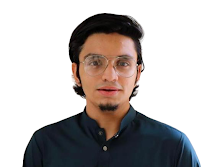
Areeb is a versatile machine learning engineer with a focus on computer vision and auto-generative models. He excels in custom model training, crafting innovative solutions to meet specific client needs. Known for his technical brilliance and forward-thinking approach, Areeb constantly pushes the boundaries of AI by incorporating cutting-edge research into practical applications, making him a respected developer in folio3.