Introduction:
Artificial Intelligence (AI) keeps reshaping industries at an unprecedented pace, with generative AI at the forefront of this transformation.
According to market analysts, the worldwide generative AI market is projected to exceed $50 billion with the aid of 2025, developing at a compound annual growth rate (CAGR) of 35%.
This surge underscores the growing adoption of AI-powered equipment throughout sectors, including healthcare, finance, training, and leisure.
Open-source generative AI models make advanced technology more accessible. Unlike proprietary options, these models enable developers, researchers, and organizations to use existing AI capabilities without high costs or licensing restrictions. The benefits encompass:
Accessibility:
Open-source models are freely available, allowing innovation across diverse domains.
Transparency:
Users gain complete visibility into the version’s structure and schooling information.
Collaboration:
A global network of contributors usually improves that equipment.
Customization:
Organizations can fine-tune models to meet unique necessities.
This article discusses the ten best open-source generative AI models of 2025, categorized by way of their primary features—textual content era, picture technology, code generation, and multimodal applications. Each version is evaluated for its unique strengths, packages, and impact on advancing AI adoption worldwide.
10 Best Open-Source Generative AI Models in 2025
Text Generation
1. GPT-NeoX
Developed by using EleutherAI, GPT-NeoX is a strong opportunity to proprietary models like GPT-3. Built on an open-supply framework, this version is highly bendy and applicable for numerous natural language processing (NLP) responsibilities.
Key functions consist of:
- Coherent and Contextually Relevant Text Generation:Â GPT-NeoX is excellent in generating human-like textual content, making it ideal for chatbots, content introduction, and virtual assistants.
- Information Summarization:Â The version approaches and condenses large statistics sets correctly, imparting concise outputs without dropping context.
- Sentiment Analysis:Â By decoding textual records, it identifies trends and emotional tone, assisting organizations in client remarks analysis.
GPT-NeoX also supports fine-tuning, making it adaptable for instructional studies, company programs, and specialized use cases.
2. Bloom
Created by the BigScience initiative, Bloom stands proud as a multilingual version assisting over 50 languages. It democratizes AI by making sure inclusivity and accessibility for global researchers. Its specific competencies encompass:
- Low-Resource Language Support:Â Bloom generates text in underrepresented languages with incredible precision.
- Diverse Multilingual Tasks: Whether it’s text translation or culturally nuanced textual content generation, Bloom grants superb performance.
- Open Access:Â This version is freely available for research, fostering innovation and collaboration.
Bloom’s layout helps language equity in AI, making it a cornerstone for inclusive generation advancements.
3. OPT (Open Pretrained Transformer)
Developed by means of Meta, OPT is an excessive-acting alternative to GPT-3 designed in particular for massive-scale text era and studies. Salient features encompass:
- Complex NLP Tasks:Â OPT handles tasks like machine translation, query answering, and content summarization with precision.
- Transparent Architecture:Â It lets in reproducible research, enabling builders to recognize and enhance its internal workings.
- Developer–Friendly Documentation:Â Comprehensive guides make certain ease of implementation for varied initiatives.
By combining transparency and performance, OPT bridges the distance among proprietary gear and open-supply models.
4. T5 (Text-to-Text Transfer Transformer)
Google’s T5 redefines NLP by converting all tasks into a text-to-textual content layout. This revolutionary method simplifies packages like translation, summarization, and query answering. Its key attributes are:
- Unified Framework:Â Treating numerous NLP obligations uniformly complements efficiency and consistency.
- Pretrained Checkpoints:Â T5 gives full-size resources for instant use across numerous domain names.
- Versatility: From simple responsibilities to complex problem-solving, T5 adapts smoothly, sponsored via Google’s strong research environment.
Image Generation
5. Stable Diffusion
Developed with the aid of Stability AI, Stable Diffusion has become synonymous with the amazing photo era from textual prompts. Its talents encompass:
- Artistic Image Creation:Â The model produces stunning, creative visuals customized as per the needs of the individual.
- Realistic Scene Generation:Â Text descriptions are converted into reasonable pictures, making it precious for industries like advertising and gaming.
- Customization:Â Users can fine-tune and rack the version for specific creative or branding initiatives.
With its accessibility and performance, Stable Diffusion is a favorite among both professionals and hobbyists.
6. DeepArt
DeepArt focuses on neural style transfer, permitting customers to apply inventive styles to regular photos. Its standout features are:
- Artistic Transformations:Â Ordinary snap shots are converted into artwork harking back to famous painters.
- Creative Experimentation:Â Designers can explore precise visual styles effortlessly.Â
- User-Friendly Interface:Â Its open-source design and intuitive gear appeal to virtual artists and educators alike.
DeepArt’s awareness on creativity guarantees its continued relevance in the virtual artwork and design groups.
Code Generation
7. CodeGen
CodeGen is tailored for software program builders, helping with code technology and of entirety throughout a couple of programming languages. Its strengths include:
- Broad Language Support:Â CodeGen understands and writes in popular languages like Python, Java, and JavaScript.
- Efficient Development:Â It completes partially written code, rushing up development cycles.
- Error Reduction:Â By presenting correct pointers, it minimizes bugs and improves coding performance.
8. Polycoder
An open-source competitor to GitHub Copilot, Polycoder excels in areas of interest programming obligations.
Key benefits are:
- Niche Language Expertise:Â Polycoder offers enhanced support for less common programming languages.
- Community Contributions:Â Its open-source nature ensures consistent enhancements driven by developers all around the globe.
- Transparency: Unlike proprietary equipment, Polycoder’s architecture is open for inspection and modification.
Audio/Multimodal Models
9. Whisper
Developed by OpenAI, Whisper is a revolutionary speech-to-textual content version. Its numerous packages include:
- Accurate Transcription: Whisper converts audio to text throughout more than one language with high fidelity.
- Real-Time Recognition:Â It recognizes speech in real time, aiding accessibility tools.
- Multimedia Integration:Â Whisper smoothly fits into video enhancing, podcast production, and accessibility answers.
By enhancing accessibility, Whisper has sizable societal and technological implications.
10. DALL·E Mini (Craiyon)
Previously known as DALL·E Mini, Craiyon generates pictures from textual descriptions.
Key capabilities include:
- User-Friendly Interface:Â Non-technical users can easily and feasibly create visuals from activities.
- Diverse Applications:Â From academic equipment to advertising campaigns, Craiyon is exceptionally versatile.
- Community–Driven Updates:Â Continuous improvements ensure its reliability and relevance.
Pros of Open Source Generative AI Models
1. Data Control
They give businesses full control over the data they collect and use. This is especially important for companies that are highly sensitive to data privacy. For instance, healthcare organizations deal with sensitive patient information, which requires strict security measures.
Open-source generative AI solutions offer secure and proper use of this data for health-related purposes, ultimately strengthening patient trust. It offers similar benefits for data control in other sectors like environmental research and finance.
2. Affordability
Building an AI system from scratch requires a significant investment of resources, which may cause companies to lose money on investment and integration of generative AI services. Plus, it demands time, money, and specialized talent.
In contrast, open-source generative AI models provide businesses with an affordable alternative that can still produce high-quality results without breaking the bank. This makes it easier for smaller businesses to explore and experiment with AI technology.
Take the e-commerce industry, for example. It integrates advanced AI without the heavy investment typically required for developing proprietary systems, allowing it to innovate and stay competitive with minimal financial strain.
3. Flexibility
Flexibility and time management are crucial concerns for tech companies. Evaluating open-source generative models provides developers with the ability to easily adapt and modify the code according to their specific requirements.
This is beneficial for tech companies like IBM to quickly adapt and customize AI tools to their specific project needs. This flexibility significantly enhances efficiency and productivity, especially in developing innovative software solutions.
4. Collaboration and Innovation
Open-source AI encourages collaboration and innovation among businesses. Companies can contribute to the open-source generative AI models evaluation to share their expertise and ideas with others in the industry. This leads to the constant improvement and evolution of AI technology.
The collaborative nature of open-source AI models significantly fuels innovation in academic and research-oriented companies like DeepMind. DeepMind primarily focuses on AI research and its applications in various scientific domains that benefit greatly from the shared advancements in open-source AI.
5. Versatility and Personalization
Open-source generative AI services are versatile and can be used in a variety of business applications such as customer service, marketing, and data analysis. It also offers assistance to fit the specific needs of each business, making it a valuable tool for any industry.
Open-source AI’s adaptability is especially helpful for marketing-related businesses like HubSpot. Known for its all-inclusive software for sales, marketing, and customer support, HubSpot leverages open-source AI for various functions, including analyzing customer behavior and creating tailored advertising campaigns.
Cons of Open Source Generative AI Models
Although it has many benefits, there are some drawbacks to using open-source generative AI within business models. They are as follows:
1. Resource Intensity
Open-source generative AI can be unreliable and require a lot of training with large amounts of data. It may not be a useful platform for all users, especially for smaller businesses like local startups or boutiques. Such companies frequently struggle with extensive data training demands of open-source AI models, as their resources are limited.
2. Limitations in Customizability
While open-source AI models are flexible, they might not always fulfill the specialized needs of all businesses. Corporations in specialized or narrow markets, like aerospace engineering, may discover that open-source AI models fall short of their intricate, sector-specific needs.
3. Lack of Support
Due to their community-driven nature, open-source AI models lack a specific support mechanism, which presents a challenge for businesses that significantly depend on technical assistance for their AI operations. A company like Salesforce, known for its customer relationship management (CRM) services, could face difficulties in this aspect.
Salesforce’s extensive use of AI to enhance customer experience and business processes means they require consistent and reliable technical support to ensure smooth operation.
4. Security Risks
The connectivity of technology to the internet, including open-source AI models, brings inherent security risks. Companies must implement robust measures to safeguard their data and infrastructure against cyber threats. An organization specializing in networking and cybersecurity solutions, such as Cisco Systems, must be especially watchful in this area.
Due to Cisco’s responsibility for managing extensive networks and protecting sensitive data, incorporating open-source AI into their systems requires a heightened emphasis on security.
5. Variable Quality
The quality and accuracy of open-source AI technology can indeed vary significantly, which impacts the reliability of business operations. A company like Adobe, renowned for its creative and digital marketing software, faces challenges with open-source AI in this context.
Adobe’s suite of products relies heavily on precision and high-quality output. If the open-source AI models they employ are built with code or data of varying quality, it could affect the performance and reliability of their software solutions.
Examples Of Open-Source Generative AI Models
While open-source generative AI models have numerous benefits, businesses need to be aware of their drawbacks. A company hoping to integrate open-source AI into its workflow should first assess its needs and available resources.
Let’s explore a few examples of open-source generative AI foundation models and their functionalities.
1. BERT
BERT, which stands for Bidirectional Encoder Representations from Transformers, is an open-source GEN-AI model of Google, like TensorFlow. It uses a natural language processor and is specifically designed to understand the context of words in sentences and improve the performance of companies in tasks like language understanding, sentiment analysis, and answering questions.
The main benefit of BERT is its cost, which lets companies use innovative language processing techniques without having to make large investments.
2. DALL·E
Another popular open-source platform is DALL·E, which is known for creating images from textual descriptions. It exhibits a remarkable capacity for understanding and visualizing ideas as well as constructing the connections between the many elements in a picture.
The realistic and imaginative outputs of DALL·E demonstrate its versatility and personalization capabilities, making it a valuable asset for creative industries that require customized visual content.
3. GPT-3.5
GPT-3.5 is an open-source language processing artificial intelligence platform that can generate human-like text. This model has been used in various applications such as chatbots and automated content creation.
It is a useful tool for companies trying to improve customer satisfaction and expedite processes. This generative AI tool’s flexibility allows companies to tailor it to their specific operational needs.
Each of these open-source generative AI models marks a notable advancement in their respective fields, highlighting the diverse potential they offer to various business models.
Conclusion
Open-source generative AI models are democratizing entry to modern AI capabilities. From text and photo generation to code assistance and audio processing, these tools empower people and businesses to innovate without boundaries. Choosing open-source answers ensures transparency, customization, and the help of a collaborative network using non-stop development.
By embracing those tools, builders, and corporations can liberate unprecedented opportunities for growth and creativity in 2025 and beyond.
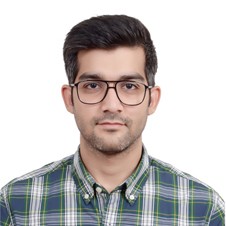
Dawood is a digital marketing pro and AI/ML enthusiast. His blogs on Folio3 AI are a blend of marketing and tech brilliance. Dawood’s knack for making AI engaging for users sets his content apart, offering a unique and insightful take on the dynamic intersection of marketing and cutting-edge technology.