AI is not just a tool; it’s a transformative force in business.” – Andrew Ng (describe who he was why is his quote important)
Andrew Ng, a leading AI expert and co-founder of Google Brain, has been instrumental in making AI accessible through platforms like Coursera and DeepLearning.AI.
His quote emphasizes that AI isn’t just a tool for efficiency—it’s a revolutionary force that can reshape industries and spark innovation. It’s a reminder for businesses to think beyond automation and embrace AI as a driver of real transformation.
Andrew Ng’s perception highlights the prominent role of AI foundation models in today’s corporate landscape. These large-scale, pre-educated models feature versatile building blocks for various applications, enabling groups to process vast records efficiently.
 In reality, over 70% of groups report that AI foundation models enhance their decision-making capabilities, using significant operational improvements.
Customization of these models permits organizations to tailor AI solutions to their particular needs. Techniques such as fine-tuning, prompt engineering, etc., empower companies to adapt these models for particular demanding situations, maximizing their effectiveness.
This degree of adaptability is crucial for preserving a competitive edge in a modern, fast-paced market.
Transforming AI into commercial enterprise experts’ methods leverages customized models to force innovation and performance.
Businesses can free up new insights and substantially improve clients’ stories by integrating AI foundation models.Â
As we explore this blog, you’ll discover unique techniques that could redefine your approach to AI and increase your business’s overall performance.
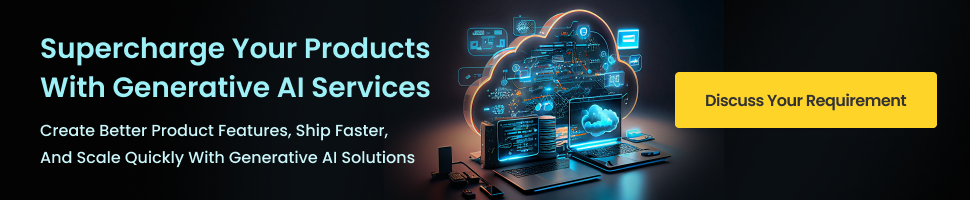
What are AI Foundation Models
AI foundation models can be understood as advanced machine learning tools. These complex models are trained on vast data sets and can perform multiple tasks.
Another name for these models is large X models (LxM). They are designed to support several AI applications, including Natural Language Processing, Computer Vision, and more. They seek vast data that can broadly be understood and later optimized for specific cases.
The terminology ‘foundation model’ encompasses a wide area of attention as far as AI is concerned. It covers many models that have been trained on large datasets employing self-supervised learning, allowing for adaptation to different downstream tasks.
The most prominent models that should be mentioned are the GPT models developed by Open AI, the BERT model developed by Google, and the DALL-E model for image generation.
The availability of such models has changed how AI systems are designed by enabling rapid deployment across different domains.
Foundation models are readily adaptable and scalable, possessing all the necessary predefined elements and being flexible.
They find application in, among other areas, writing content or pictures as they can work with vast amounts of complex data.
The specific design of the given model enables companies to save time and money since they do not have to begin development from scratch but rather build on what has already been trained.
As AI is a growing entity, it is anticipated that the importance of foundation models will profoundly transform various industries, including healthcare.
With the evolution of AI, the significance of these AI models will reach its peak, which can be handy for users in the following ways:
Techniques for Customization
1- New Domain Knowledge or Continued pretraining
The other form of continued pretraining involves retraining the foundation model on a more specific data set pertinent to the chosen application tasks.
In this way, it builds on its core understanding of language and tasks on the sub-domain level. It plays a major role when a large amount of domain-specific data is available.
2- Fine-Tuning
Overfitting models for every new user is often ineffective, and fine-tuning can do the job. Fine-tuning more accurately adjusts the model’s weights according to a smaller training set related to the new task.
Fine-tuning can narrow down the focus of the foundation model and allow it to look only for certain aspects related to the given context.
Works particularly well for many tasks requiring high precision – such as automated sentiment analysis or customer support.
3- Prompt Engineering
Prompt engineering focuses on understanding user context and creates effective prompts that direct the model and limit its response scope.
With examples that contain context, people can obtain the results they desire without having to change the weights.
Prompt engineering is usually the next step after prompting, with the aim of altering foundation models for use on specific tasks.
4- Few Shot and Zero-Shot Learning
Few-shot and zero-shot learning are two increasingly popular model technologies that allow us to apply a model to a task with few or no relevant prior examples.
Few-shot learning uses few annotated samples, while zero-shot learning uses common sense knowledge without specific knowledge.
5- Adapter Layers
Adapter layers are additional lightweight modules integrated into an existing pre-trained model that enables fine-tuning for a given task without retraining the full model architecture.
This method preserves the original model while significantly increasing its applicability.
6- Domain Adaptation
Domain adaptation involves fine-tuning a foundation model on data relevant to the domain in which it will operate to boost its performance.
Tailoring the pre-training phase using data from specific domains guarantees that the model comprehends industry-related vocabulary and contextual references.
This leads to more effective performance in domains like finance and health care.
7- Knowledge Distillation
Knowledge distillation involves transforming knowledge from a larger, complex model to a smaller, more efficient one.
This procedure preserves crucial talents while decreasing computational requirements, making it suitable for deployment in aid-constrained environments.
8- Reinforcement Learning with Human Feedback (RLHF)
RLHF combines reinforcement learning with human feedback to improve version performance iteratively. Incorporating consumer feedback into the training loop facilitates models to better align with consumer expectations and choices over time.
9- Multimodal Fine-tuning
Multimodal fine-tuning adapts models to handle multiple types of data inputs, such as text, images, and audio.
This technique broadens the model’s applicability to diverse tasks and industries, making it versatile in real-world applications.
10- Pre-pending Contextual Information
Pre-pending contextual records include applicable information before the main input to guide the model’s understanding and output quality.
This approach can appreciably enhance reaction accuracy by providing essential context in advance.
11- Augmented Training with Synthetic Data
Augmented education uses artificial data generated through diverse methods to complement actual datasets.
This method can enhance a model’s strength and performance, mainly when real-world facts are constrained or tough to obtain.
Implementing Customization with Folio3
Folio3 specializes in customizing AI foundation models to meet numerous business needs. Our approach guarantees that groups can leverage AI effectively while aligning with their precise use cases.
1- Tailored Solutions
At Folio3, we understand that one size no longer matches all demands. We provide customized solutions that adapt foundation models according to your specific requirements.
This customization enhances the version’s relevance and overall performance in your particular enterprise, whether it’s healthcare, finance, or retail.
2- Expertise in Implementation
Our team of AI specialists is skilled in various customization techniques, fine-tuning, and spark-off engineering.
We guide you through the implementation system, making sure that your version is optimized for the task in a timely manner. This expertise maximizes results.
Folio3’s Step by Step Process for Customizing Foundation Models:Â
3- Data-Driven Customization
Customization starts with understanding your data. We analyze your current datasets to interpret the model’s training data.
This data-driven approach permits us to fine-tune models successfully, making sure they perform well in real-world applications.
4- Continuous Support
Folio3 provides ongoing support at some point in the customization process. From initial setup to post-deployment monitoring, we ensure that your AI answers evolve along with your business needs. Our dedication to nonstop development maintains model accuracy and relevance over time.
5- Scalable Infrastructure
We leverage strong cloud infrastructure to facilitate smooth customized model integration into your present systems. This scalability ensures that as your business grows, your AI competencies can enlarge without compromising performance.
6- Proven Track Record
With a history of successful implementations throughout numerous sectors, Folio3 has established itself as a leader in AI customization.
Our case studies demonstrate how we have transformed groups by deploying customized AI solutions that power efficiency and innovation.
By partnering with Folio3, corporations can liberate the total potential of AI foundation models, ensuring they are powerful and perfectly aligned with their operational goals.
Evaluating Model Performance
Evaluating AI foundation models is vital for ensuring their effectiveness and alignment with business needs and requirements.
A comprehensive assessment approach combines quantitative and qualitative strategies.
1- Benchmark Datasets
Using benchmark datasets, we can adopt a systematic approach to evaluating the model’s performance. These datasets offer predefined obligations and metrics to evaluate accuracy, pace, and scalability.
Key Metrics:
Key metrics for assessment encompass
- Accuracy:Â
The percentage of correct outputs produced by the model. - ROUGE:
Measures overlap in generated and referenced textual content. It is often found handy for providing a summary of the text. - BLEU:
Assesses translation quality by evaluating generated text for references.
These metrics offer a clear view of the model’s abilities.
2- Continuous Monitoring
After deployment, ongoing overall performance tracking is critical. Analyzing user interactions allows us to perceive improvement regions, making sure the model adapts to evolving needs.
3- Ethical Considerations
Addressing bias and equity is crucial in evaluation. Tools like the Foundation Model Transparency Index help track transparency in AI development, which assures that the model is built ethically and responsibly.
A sturdy assessment framework maximizes the value of AI foundation models while aligning them with strategic business objectives.
Conclusion:
Transforming AI into business specialists entails leveraging foundation models to enhance decision-making, streamline operations, and force innovation. These models function as flexible gear that may be fine-tuned for unique enterprise packages, substantially lowering improvement time and costs.
By using foundation models, businesses can automate recurring tasks, such as customer service and facts evaluation, allowing human resources to focus on more strategic tasks. This automation multiplies efficiency and productivity across various sectors, including healthcare, finance, and e-commerce.
Moreover, foundation models provide information-driven insights derived from vast datasets. This functionality allows corporations to make knowledgeable decisions based on complete analyses instead of intuition alone. As those models evolve, they promise to improve accuracy and decrease biases in decision-making methods.
However, businesses must additionally navigate demanding situations, including moral considerations and capability biases inherent in the schooling information. Addressing these issues is vital for ensuring truthful and obvious AI packages.
In conclusion, by effectively imposing and customizing foundation models, businesses can transform AI into an effective ally that enhances their operational competencies and drives sustainable growth. This transformation now positions businesses at the forefront of technological advancement and empowers them to reply dynamically to converting marketplace needs.
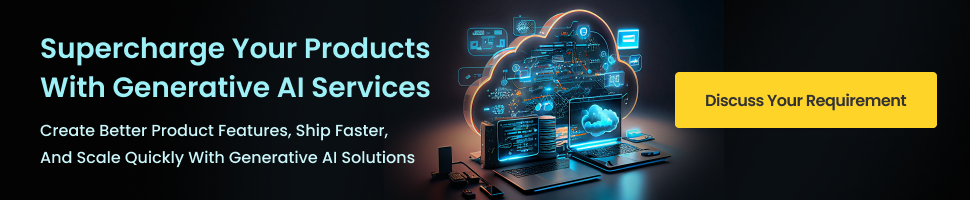
FAQs
Implementing foundation models can be tricky. Companies often struggle with integrating these models into existing systems, ensuring data privacy, and maintaining the models over time. Additionally, they must consider how to scale the solution effectively as their needs grow.
Bias can be a significant issue with foundation models since they learn from the data they’re trained on. If that data contains biases, the model might produce biased results. To combat this, organizations must actively monitor outputs and refine their datasets to promote fairness in AI applications.
Training foundation models requires some serious computational power! You’ll need high-performance GPUs and ample data storage to handle the large datasets. The proper infrastructure is crucial for successfully training and deploying these models.
Security is a big concern when working with AI models, as they can be vulnerable to cyberattacks. Organizations should implement strong security measures like access controls, regular audits, and encryption to protect sensitive data used during training and deployment.
Transfer learning is a game-changer for customizing foundation models! It allows these models to take the knowledge learned from one task and apply it to another. This means you can fine-tune a model with less task-specific data, making it easier and quicker to adapt it to your unique needs.