Executive Summary:
With innovative uses like DeepFake and ChatGPT, generative AI has become a new buzzword. Generative AI uses AI and machine learning methods to provide computers the ability to create synthetic content based on training data, including text, images, audio, and video.
The issues facing generative AI are data protection, misuse for fraudulent or illegal purposes, and delusion.
In this post, we examine generative AI models, how they function, their benefits and drawbacks, their applications, and the actions you can take to get the most out of them.
What is “generative AI”?
The technology known as generative AI models allows users to produce a new text, audio, or visual output using pre-existing materials. With generative AI, computers can identify the underlying pattern in the input and generate comparable material.
There are numerous methods, including:
- Transformers
Transformers that mimic cognitive attention, such as GPT-3, LaMDA, Wu-Dao, and ChatGPT, differentially estimate the importance of the input data portions. They receive training to comprehend the language or image, classify data, and produce words or images from enormous datasets.
- GANs, or generative adversarial networks
GANs are neural networks competing to find an equilibrium between the two: a generator and a discriminator.
- Creating new data or content resembling the source data falls to the generator network.
- The discriminator network is responsible for distinguishing between the source and generated data to determine which is closest to the original data.
- Differential auto-encoders
- The decoder recreates the original information from the compressed code that the encoder created from the input.
- This compressed representation stores the input data distribution in a considerably reduced dimensional representation if properly selected and trained.
What advantages does generative AI offer?
- Overall
As generative AI’s capabilities advance, many occupations will be affected. For example, much of modern human labor goes toward producing text, photos, and video. That has a lot of potentials to be automated.
- Identity Protection
People who do not want to reveal their identities during job interviews or other situations can be protected using generative AI avatars.
- Robotics control
Generative AI models enable reinforcement machine learning models in simulation and the real world to be more abstract and less biased.
- Healthcare
Generative AI in healthcare provides the early detection of possible malice to develop successful remedies. For instance, GANs compute several X-ray picture angles to visualize the potential tumor growth.
What difficulties does generative AI face?
- Security
Generative AI models can be used fraudulently, such as to con people.
- Overestimating capabilities
Generative AI algorithms need a huge amount of training data to complete tasks. GANs, however, cannot produce wholly original images or messages. Instead, they blend the things they have been taught in various ways. Because of this, they are known as “stochastic parrots” by some computer scientists.
- Hallucination
Some Generative AI models, such as GANs, may yield unexpected outcomes.
- Data privacy
Health-related applications raise privacy issues involving data at the individual level.
- Copyrights
Generative AI copyrights are currently the subject of legislation and legal actions.
How to Develop in the Age of AI: 7 Steps
1. Find applications for generative AI in your life or company. For example, most white-collar work involves producing new text, images, or videos. Thus there should be a wide range of activities.
2. Sort these use cases according to how well generative AI might fit them. For example, using generative AI, certain procedures frequently become simpler to automate:
- Do any pertinent texts or photos exist in private or public data that could help the model? A huge language model, for instance, wouldn’t be able to write about a brand-new technology because it wouldn’t have absorbed any material on the subject.
- Is the result unbiased or subjective? Because of concerns like hallucination, generative AI solutions can make mistakes when faced with tasks that may be assessed using objective criteria. For instance, generative AI models that solve math problems could be better.
- What are the strategies for error tolerance and mitigation? Whether the model’s output is susceptible to subjective or objective evaluation, there will be instances where the findings fall short of expectations. Humans-in-the-loop is required in such instances to alleviate the situation, but if the system must react in milliseconds, this may not be achievable.
3. By ROI, order these use cases. You can determine your top priority projects by weighing the advantages and the costs of incorporating generative AI into your business processes.
4. Create a new procedure. The new process can involve more human-in-the-loop or integrations for a straightforward procedure.
5. Decide which generative AI technology to use.
6. Conduct a pilot with precise goals and track outcomes. For instance, you might have chosen the use case for your pilot to create blog articles. You may need to consider the following questions to determine whether generative AI is advantageous:
- Are the new blog posts better or worse than previous ones in your important areas?
- What amount of work is saved?
7. Find improvements and implement the revised procedure: For instance, you might have conducted the pilot employing users who were simultaneously using an internal desktop app and an online generative AI application. Incorporating generative AI into your applications and utilizing APIs can unlock extra savings that should be considered during go-live. Screen switching might diminish productivity.
Is supervised learning used in generative AI?
GANs is a framework for semi-supervised learning that uses generative adversarial networks. Semi-supervised learning approaches use manually labeled training data for supervised learning and test dataset for unsupervised learning approaches to construct models that can generate predictions beyond the labeled data by utilizing labeled data.
There are certain benefits of Gans’s semi-supervised structure, which is an example of generative AI, over supervised learning, which includes:
Overfitting: As generative models often contain fewer parameters, overfitting is more difficult. Moreover, generative models are more resistant to occlusions because the training process involves massive amounts of data.
Human bias: Unlike supervised learning, human labels in generative modeling are less obvious. Learning is based on the characteristics of the data, which enables avoiding erroneous correlations.
Model bias: Generic models do not produce samples that resemble those in the training set. The conflict between shape and texture is thus resolved.
The last say:
As we’ve indicated, generative AI and machine learning have transformed businesses and will do so for a long time. Implementing generative AI into business contexts reduces time spent on repetitive tasks, increases staff productivity, and improves customer experience across marketing, operations, and sales. Also, it aids with error prevention and crisis detection.
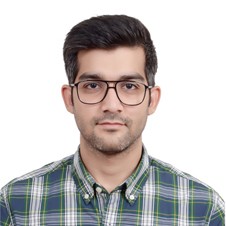
Dawood is a digital marketing pro and AI/ML enthusiast. His blogs on Folio3 AI are a blend of marketing and tech brilliance. Dawood’s knack for making AI engaging for users sets his content apart, offering a unique and insightful take on the dynamic intersection of marketing and cutting-edge technology.