Speech recognition AI, also known as automatic speech recognition (ASR), is a technology that enables machines to understand human speech and convert it into text. Since its inception in the 1950s, this form of artificial intelligence has made significant strides and is now widely used across various industries and applications. In this blog post, we’ll explore how speech recognition AI works, its uses and benefits, and the potential challenges and limitations it faces.
What Is Speech Recognition AI?
Speech recognition AI is a form of artificial intelligence that allows machines to recognize and understand human speech like humans do. It involves processing audio signals, deciphering language patterns, and converting them into text for further analysis or action. This technology uses advanced algorithms and machine learning techniques to interpret an individual’s words, phrases, and sentences.
Speech recognition AI typically involves four main stages:
- Acoustic Model: This stage involves converting recorded audio signals into digital data that the machine can recognize and process.
- Language Model: In this stage, the system uses statistical methods to analyze language patterns and predict words or phrases based on previous input.
- Pronunciation Model: This model helps the system understand different accents, dialects, and intonations to improve accuracy in recognizing speech.
- Decoder: The decoder combines information from the previous models to generate a transcription of the spoken words.
Brief History
Speech recognition technology started in the 1950s with systems that could only recognize a few words. Early examples, like IBM’s Shoebox, showed basic abilities but had a hard time with natural language. In the 1980s, hidden Markov models improved things, allowing for better recognition. Now, in the 21st century, speech recognition AI has surpassed human performance in some tasks, all possible due to the advances in deep learning and natural language processing.
How Does Speech Recognition AI Work?
Speech recognition AI converts spoken language into text. Here’s a simple breakdown of the process.
Basic Workflow
- Audio Capture: The process begins by capturing audio through a microphone or other devices like headphones or smart speakers. This audio is then converted into a digital format for processing.Â
- Feature Extraction: The captured audio is broken down into smaller units, like phonemes (the smallest sound units) and spectrograms (visual maps of sound frequencies). These features are essential for understanding the speech.
- Acoustic Modeling: Acoustic models are trained using large speech datasets to learn how audio features link to phonemes. This helps the system recognize different sounds, considering factors like the speaker’s voice, accent, and background noise.
- Language Modeling: Language models use grammar rules and vocabulary to predict the most likely words or phrases based on the context. This step improves accuracy by ensuring the recognized words make sense in the given context.
- Text Output: Finally, the system combines the insights from the acoustic and language models to produce text, converting the recognized sounds into grammatically correct sentences.
How Does Speech Recognition AI Work?
Speech recognition AI converts spoken language into text. Here’s a simple breakdown of the process:
Basic Workflow:Â
- Audio Capture: The process begins by capturing audio through a microphone or other devices like headphones or smart speakers. This audio is then converted into a digital format for processing.Â
- Feature Extraction: The captured audio is broken down into smaller units, like phonemes (the smallest sound units) and spectrograms (visual maps of sound frequencies). These features are essential for understanding the speech.
- Acoustic Modeling: Acoustic models are trained using large speech datasets to learn how audio features link to phonemes. This helps the system recognize different sounds, considering factors like the speaker’s voice, accent, and background noise.
- Language Modeling: Language models use grammar rules and vocabulary to predict the most likely words or phrases based on the context. This step improves accuracy by ensuring the recognized words make sense in the given context.
- Text Output: Finally, the system combines the insights from the acoustic and language models to produce text, converting the recognized sounds into grammatically correct sentences.
Core Technologies Behind Speech Recognition AI
Speech recognition AI relies on various technologies to deliver accurate and reliable results. Understanding these technologies helps us appreciate how speech recognition systems function.
1. Natural Language Processing (NLP)
Natural Language Processing (NLP) is a key component of AI that focuses on how computers interact with human language. In the realm of speech recognition, NLP is vital for grasping the context and meaning of spoken words. It aids in several important tasks:
- Grammar and Syntax: NLP identifies relationships between words by analyzing sentence structures, enabling clearer communication.
- Semantics: This aspect helps the system understand the meanings behind words and phrases, allowing for more accurate interpretations.
- Pragmatics: NLP also considers language context, such as speaker intent and social cues, which enhances understanding.
When combined with speech recognition, NLP techniques enable systems to process human language more naturally and precisely.
2. Machine Learning and Deep Learning
Machine learning, a subset of AI, allows systems to learn from data without needing explicit programming. In speech recognition, machine learning algorithms train models on extensive speech datasets. Deep learning, a specialized form of machine learning, has transformed this field by allowing systems to identify complex patterns.
- Feature Extraction: Algorithms extract significant features from audio signals, such as pitch and intensity, which are crucial for recognizing speech.
- Model Training: Deep learning models, like neural networks, learn from large datasets to recognize speech patterns and produce accurate transcriptions.
- Continuous Improvement: As these algorithms are exposed to more data, they adapt and enhance their performance over time.
3. Neural Networks
Neural networks, inspired by the human brain, consist of interconnected layers of nodes that process information. In speech recognition, specific neural network types have shown notable effectiveness:
- Recurrent Neural Networks (RNNs): RNNs excel at processing sequential data, making them ideal for capturing the temporal relationships between words, which boosts accuracy.
- Convolutional Neural Networks (CNNs): CNNs are adept at handling grid-like data, such as images, and can be applied to spectrograms in speech recognition to extract relevant features.
By integrating RNNs and CNNs, we can create hybrid models that achieve exceptional performance in understanding speech.
4. Big Data and Training Models
The success of speech recognition systems greatly depends on the quality and volume of training data. Amassing vast amounts of diverse speech data is essential for developing robust models. Key steps include:
- Data Collection: Gathering a wide range of speech samples, including different accents, dialects, and noise conditions, ensures models can adapt to real-world variations.
- Data Preprocessing: This step involves cleaning the data to remove noise and inconsistencies, which is crucial for effective training.
- Model Training: The preprocessed data is then used in iterative learning processes to train neural networks.
- Continuous Improvement: As new data becomes available, the models can be retrained, leading to continuous enhancement in accuracy and performance.
By understanding these interconnected components, we gain insight into how speech recognition AI operates and evolves.
 Advantages and Challenges of Speech Recognition AI
 Advantages
- Convenience and Efficiency: Speech recognition makes tasks easier by letting you talk instead of typing, boosting productivity. You can dictate emails or documents without stopping to type, saving time. It also helps automate tasks like setting reminders or searching online, making your personal and work lives more efficient.
- Increased Accessibility: Speech recognition has greatly helped people with disabilities or language barriers. For example, converting speech to text allows those with hearing impairments to join conversations. It also supports different languages, helping non-native speakers and those with limited literacy skills engage in education and work, promoting inclusivity.
Challenges
- Accuracy and Misinterpretation: Despite improvements, speech recognition can still struggle with accuracy, especially with accents, dialects, and background noise. Misunderstandings can occur in important situations like healthcare or legal settings, and errors can happen if the technology doesn’t fully understand what the user means.
- Privacy Concerns: Speech recognition systems collect sensitive user data, raising privacy concerns. Users worry about storing, sharing, or using their voice recordings. Strong data protection and clear user policies are essential to build trust, especially in sensitive fields like healthcare and finance.
- Dependency on High-Quality Data: For speech recognition AI to work well, it needs a lot of diverse training data. Limited data can lead to biased or inaccurate results, especially with different accents or languages. It’s important to collect high-quality data that reflects real-world situations and to continuously update these data sets to keep up with changing language patterns and user needs.
The Future of Speech Recognition AI
-
 Emerging Trends
The future of speech recognition AI aims to make conversations with machines feel more natural. As AI advances, it will better understand context, tone, and emotions, leading to smoother and more friendly interactions. It will also support multiple languages, making global communication easier, and will learn from users’ past interactions to personalize experiences.
-
 Potential Impact on Industries
As speech recognition technology improves, it will transform various industries. Education could offer personalized learning experiences that adapt to each student’s needs. In entertainment, voice commands could revolutionize how we interact with content, like searching for shows or playing games. Advanced voice recognition might help analyze witness statements in law enforcement in real time, leading to more accurate suspect profiles.
-
Integration with Other Technologies
Combining speech recognition with technologies like the Internet of Things (IoT) and augmented reality (AR) opens up exciting possibilities. Imagine controlling smart home devices just by speaking or navigating AR environments using voice commands. In healthcare, speech recognition could integrate with AI to help providers access patient data and visualize treatments, making everyday tasks easier and more enjoyable.
Conclusion
Speech recognition AI has come a long way and will only get better. It’s making things more accessible and convenient for everyone, and the impact on different industries could be huge. However, we must still address challenges like accuracy, privacy, and data dependency before it can be widely adopted. As speech recognition technology combines with other technology, we can expect even more advancements to make our lives easier and more connected.Â
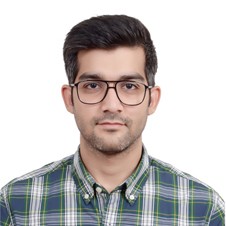
Dawood is a digital marketing pro and AI/ML enthusiast. His blogs on Folio3 AI are a blend of marketing and tech brilliance. Dawood’s knack for making AI engaging for users sets his content apart, offering a unique and insightful take on the dynamic intersection of marketing and cutting-edge technology.