Introduction
AI has moderately been used in many health practices, especially in pharmaceuticals. With millions of diseases out there, producing drugs quickly is challenging. But now, with AI-driven tools, navigating those complex datasets is much easier.
This means pharmaceutical companies can research, develop, and bring their solutions to market much faster.
So, not only does AI speed up the process from lab to market, but it also makes tailored treatments possible, giving us hyper-personalized therapies that fit individual needs.
To get a more apparent concept, look at perspectives from the healthcare sector on AI in pharmaceuticals.
Pfizer: “AI has the potential to accelerate our drug discovery process significantly. By utilizing advanced algorithms to sift through large datasets, we can identify promising drug candidates more efficiently than ever before.” — Dr. Michael Dolsten, Chief Scientific Officer, Pfizer.
Novartis: “AI is reshaping how we approach patient treatment and clinical trials. By harnessing AI, we can better understand patient needs and develop more personalized therapies, which is at the heart of our mission.” Vas Narasimhan, CEO, Novartis
Roche: “Integrating AI into our workflows allows us to leverage data like never before. It empowers our researchers to make informed decisions quickly, potentially saving years in the drug development timeline.” Severin Schwan, CEO, Roche.
For a more in-depth insight, continue reading this blog, which explores the opportunities and complexities of AI in the pharmaceutical sector.
It will also highlight how intelligent technologies are reshaping the industry, supported by case studies, practical examples, and insights into future trends. Before that, let’s start with the basics.
AI in Pharmaceuticals: A Comprehensive Introduction
AI in pharmaceuticals represents a notable convergence of technology and healthcare, fundamentally changing how drugs are discovered, developed, and delivered.
Beyond the well-known applications of AI—like speeding up drug discovery and personalizing treatment plans—what’s truly unique is its ability to redefine collaboration among various stakeholders in the pharmaceutical ecosystem.
Using AI-driven platforms, pharmaceutical companies can foster more dynamic interactions between researchers, clinicians, and patients.
This creates a feedback loop that not only revamps real-world applications but also ensures that the voices of all stakeholders are heard, ultimately leading to more effective and targeted therapies.
Furthermore, AI tools used in pharmaceuticals can aid in predicting future healthcare trends, allowing pharmaceutical companies to proactively adapt their strategies in response to emerging health challenges.
This capacity for real-time adaptation and collaboration positions AI not just as a tool for efficiency but as a catalyst for innovation and improved health outcomes across the board.

The Increased Demand for AI in Pharmaceuticals Stats
To see the increasing impact of AI in pharmaceuticals, look at the following industry forecasts; global AI in the pharmaceutical market is set to experience remarkable growth, with a projected compound annual growth rate (CAGR) of around 42.68%.
By 2025, the market is expected to reach an estimated value between $350 billion and $410 billion. This explosive growth reflects the increasing recognition of AI’s potential to enhance productivity, reduce costs, and improve patient outcomes across the pharmaceutical landscape.
As companies integrate AI technologies, they are streamlining operations and also introducing approaches in therapies that meet evolving healthcare needs.
Applications of AI in Pharmaceuticals
These applications illustrate how AI is influencing the pharmaceutical landscape, driving innovations that lead to more effective treatments and improved patient outcomes.
1- Drug Discovery and Development
AI speeds up the drug discovery process by comprehending huge chemical libraries and predicting how effective and safe potential drug candidates might be.
As machine learning algorithms are multifaceted, they can go through extensive datasets to find promising compounds, which lessens costs compared to traditional drug development methods.
AI also forecasts how different molecules will interact with biological targets, which allows researchers to zero in on the most viable candidates right from the start. This leads to quicker clinical trials and, ultimately, more effective therapies.
2. Personalized Medicine
AI is remarkable for personalized medicine; it designs treatment plans based on individual patient data. By analyzing genetic information, medical histories, and lifestyle factors, AI algorithms can identify optimal therapies for specific patients.
This approach improves treatment efficacy and minimizes adverse effects by ensuring that patients receive medications that align with their unique biological profiles.
The integration of AI in personalized medicine represents a shift from one-size-fits-all treatments to more customized healthcare solutions.
4. Predictive Analytics for Disease Prevention
AI is increasingly used for predictive analytics to identify individuals at high risk for certain diseases. AI can forecast disease onset and recommend preventive measures by inspecting patient data patterns, including genetic predispositions and lifestyle choices.
This proactive approach allows healthcare providers to implement targeted interventions before diseases manifest, ultimately improving population health outcomes [
5. Real-Time Patient Monitoring
The adoption of wearable technology in pharmaceuticals, powered by AI, allows for real-time monitoring of patient health metrics.
These devices, equipped with advanced AI algorithms, continuously gather data on vital signs and activity levels. If any abnormalities are detected, they can quickly alert healthcare providers for immediate intervention.
This ongoing feedback loop improves patient management beyond traditional clinical settings, enabling timely interventions and improving overall care.
6. Clinical Decision Support Systems (CDSS)
.AI-driven Clinical Decision Support Systems (CDSS) empower healthcare professionals by quickly analyzing patient data to inform treatment decisions.
These systems integrate genetic information with clinical findings, providing personalized recommendations that align with each patient’s unique health profile.
For example, platforms like xDECIDE leverage AI to deliver tailored cancer treatment options based on real-world evidence and intricate genetic data.
This approach enhances diagnostic accuracy and streamlines treatment planning, ultimately improving patient outcomes.
Challenges That Occur in Pharmaceuticals
The introduction of AI technologies in the pharmaceutical industry has streamlined multiple stages of its operations.
However, achieving success requires careful attention to several challenges and considerations. Let’s investigate these points in depth.
1. Lack of Data Availability
One of the primary challenges facing the integration of AI in pharmaceuticals is the lack of sufficient and high-quality data.
AI algorithms thrive on large datasets to learn patterns and make predictions. However, in the pharmaceutical sector, many diseases have limited patient populations, resulting in smaller datasets that are often insufficient for training robust AI models.
This scarcity complicates the development of reliable predictive models, leading to potential inaccuracies and biases in drug discovery and clinical trial outcomes.
2. Data Bias and Representation
Another significant hurdle is data bias, which can arise from unrepresentative datasets. Historically, certain demographic groups have been underrepresented in medical research, leading to AI models that may need to generalize better across diverse populations.
This lack of inclusivity can result in misdiagnoses or ineffective treatments for marginalized groups. Addressing this issue requires a concerted effort to ensure that datasets are comprehensive and representative, which is both a logistical and ethical challenge for pharmaceutical companies.
3. Ethical Concerns
The ethical implications surrounding AI use in pharmaceuticals cannot be overlooked. As AI systems begin to influence clinical decision-making, questions about accountability and transparency arise.
It becomes crucial to understand how AI-generated recommendations are made and who is responsible for any adverse outcomes.
Furthermore, ethical concerns about patient privacy and data security must be prioritized to maintain public trust in AI technologies within healthcare settings.
4. Regulatory Challenges
Navigating the regulatory landscape presents another challenge for the adoption of AI in pharmaceuticals.
The industry is heavily regulated, requiring strict adherence to guidelines that can slow down the implementation of innovative technologies like AI.
Companies must collaborate with regulatory bodies to develop frameworks that allow for the safe and effective use of AI while ensuring compliance with existing laws.
This process can be time-consuming and may hinder the rapid advancement of AI applications in drug development.
5. Integration with Traditional Methods
Finally, the integration of AI with traditional experimental methods poses a significant challenge. While AI has shown promise in accelerating drug discovery and optimizing clinical trials, it often needs to be used alongside conventional techniques to validate findings and ensure safety.
This hybrid approach requires a shift in mindset within pharmaceutical companies, as they must balance innovation with established practices.
Developing effective strategies for this integration is essential for maximizing the benefits of AI while maintaining rigorous scientific standards.
Benefits Of AI in Pharmaceuticals
1. AI-Driven Drug Repurposing
Artificial Intelligence facilitates the repurposing of existing pharmaceuticals by meticulously analyzing extensive datasets derived from clinical trials and patient records.
This accelerates the identification of novel therapeutic applications, significantly minimizing development timelines and associated costs, thereby expediting patient access to innovative treatments while optimizing the utilization of current pharmaceutical assets.
2. Synthetic Data Generation for Research
AI proficiently generates synthetic data to augment scarce real-world datasets, addressing challenges related to data insufficiency, privacy concerns, and regulatory compliance.
By simulating diverse patient populations and treatment outcomes, AI models produce high-quality datasets that researchers can employ for hypothesis testing, algorithm training, and enhancing clinical trial design, facilitating robust analyses without compromising patient confidentiality.
3. Real-Time Pharmacovigilance
AI enhances pharmacovigilance by enabling the real-time surveillance of drug safety and efficacy in the post-market phase.
Leveraging natural language processing (NLP) and machine learning, AI can scrutinize social media, online forums, and electronic health records to swiftly identify adverse drug reactions (ADRs), allowing pharmaceutical entities to respond promptly to safety concerns and thereby improving patient protection and regulatory adherence.
4. Supply Chain Resilience through AI Forecasting
AI fortifies supply chain resilience by employing sophisticated forecasting models that take into account global disruptions, such as pandemics and geopolitical tensions.
By analyzing historical data and real-time inputs, AI can anticipate supply chain variations and potential bottlenecks, enabling companies to formulate contingency plans that mitigate the risks of drug shortages and ensure the continuous availability of critical medications during crises.
5. Augmented Reality (AR) for Training and Education
AI-driven augmented reality has the potential to transform training and education within the pharmaceutical sector.
AR applications can simulate intricate drug mechanisms, patient interactions, and clinical scenarios, providing immersive, experiential learning opportunities for healthcare professionals and pharmaceutical sales teams.
This enhancement of comprehension through interactive visualizations and real-time feedback ultimately leads to improved retention of vital information and better patient outcomes.
Case Studies of AI in Pharmaceuticals
The pharmaceutical industry showcases a range of case studies featuring AI models in action. Let’s examine and select these examples.
1. AstraZeneca and DeepMind
AstraZeneca, a global biopharmaceutical company, partnered with DeepMind, a leading artificial intelligence research lab, in 2016.
DeepMind’s AI technology was used to predict the 3D structure of proteins, a crucial step in drug discovery.
The AI-powered system accurately predicted the 3D structures of more than 100 million proteins, significantly accelerating the drug discovery process and potentially leading to the development of new treatments for diseases like Alzheimer’s and Parkinson’s.
2. GSK and Exscientia
GlaxoSmithKline (GSK), a major pharmaceutical company, partnered with Exscientia, a drug discovery company focused on AI, in 2015.
Exscientia’s AI platform was used to design and optimize new drug molecules, reducing the time and cost of drug discovery.
GSK and Exscientia have successfully identified and progressed several drug candidates using their AI-powered approach, demonstrating the potential of AI to revolutionize the pharmaceutical industry.
3. IBM Watson Health and Merck
IBM Watson Health, a division of IBM focused on healthcare, partnered with Merck, a global pharmaceutical company, in 2016.
IBM Watson Health’s cognitive computing platform was used to analyze vast amounts of medical data and identify potential drug targets.
The partnership has led to the discovery of new drug candidates for cancer and other diseases, highlighting the power of AI to accelerate drug discovery and improve patient outcomes.
Some of the AI Models Used in Pharmaceuticals
Here are five famous models used for AI in pharmaceuticals, along with brief descriptions:
1. DeepChem
DeepChem is an open-source library that uses deep learning to facilitate drug discovery. It provides tools for molecular modeling and allows researchers to predict the properties of chemical compounds, streamlining the identification of potential drug candidates.
2. AlphaFold:
Developed by DeepMind, AlphaFold uses AI to accurately predict protein structures. Understanding protein structures is crucial for drug design, as it helps identify how drugs can interact with their targets.
3. Chemoinformatics Models:
These models leverage machine learning algorithms to analyze chemical data and predict molecular activity. By identifying relationships between molecular structures and biological effects, they assist in drug discovery and optimization.
4. BiLSTM (Bidirectional Long Short-Term Memory):
This neural network model is effective for sequence prediction tasks, such as analyzing patient health records. In pharmaceuticals, BiLSTM can be used to predict patient responses to treatments based on historical data.
5. Generative Adversarial Networks (GANs):
GANs are used to generate new molecular structures by training two neural networks against each other. In pharmaceuticals, they help in discovering novel drug candidates by creating diverse and viable chemical compounds.
Navigating Pharma Challenges with Folio3’s AI Innovations
We stand as a transformative partner in the pharmaceutical industry, delivering leading-edge AI healthcare solutions that address its most complex challenges. What sets our AI models apart is the frictionless integration of cognitive machine learning and advanced computer vision.
This powerful combination transforms the drug discovery process, empowering researchers to navigate vast datasets efficiently and identify potential drug candidates with remarkable precision and speed.
Conclusion
Artificial intelligence is genuinely making progress in the pharmaceutical industry. We’re seeing faster drug discovery processes, advancements in personalized medicine, and improved patient monitoring. While there are challenges, like data limitations and regulatory hurdles, companies like Folio3 are pioneering ways to overcome them with innovative AI solutions.
As the industry adapts to these new technologies, we can expect faster, safer, and more effective patient treatments. Ultimately, the future will be a collaborative effort between AI and human expertise, leading to continuous improvements in drug development and patient care.
Embracing these changes is vital for success in today’s competitive landscape.

FAQS
AI is primarily used in drug discovery and development, personalized medicine, clinical trials optimization, drug repurposing, predictive analytics for patient outcomes, and in managing supply chains. It helps in analyzing large datasets to identify potential drug candidates and streamline the research process.
AI improves drug discovery by enabling the analysis of vast amounts of biological and chemical data to identify new drug candidates more quickly and efficiently. Machine learning algorithms can predict how different compounds will interact with biological targets, reducing the time and cost associated with traditional laboratory experiments.
AI can enhance clinical trials by improving patient recruitment through better identification of suitable candidates, optimizing trial designs, monitoring patient data in real-time for safety and efficacy, and analyzing results more rapidly. This leads to faster and more efficient trials, ultimately bringing drugs to market sooner.
Challenges include data privacy concerns, the need for high-quality and standardized data, integration of AI systems with existing processes, regulatory hurdles, and the potential for biases in AI algorithms. Additionally, there is a need for skilled professionals who can work with AI technologies.
AI contributes to personalized medicine by analyzing patient data, including genetics, lifestyle, and medical history, to tailor treatments to individual patients. This approach enhances treatment efficacy and minimizes adverse effects by matching patients with the most suitable therapies.
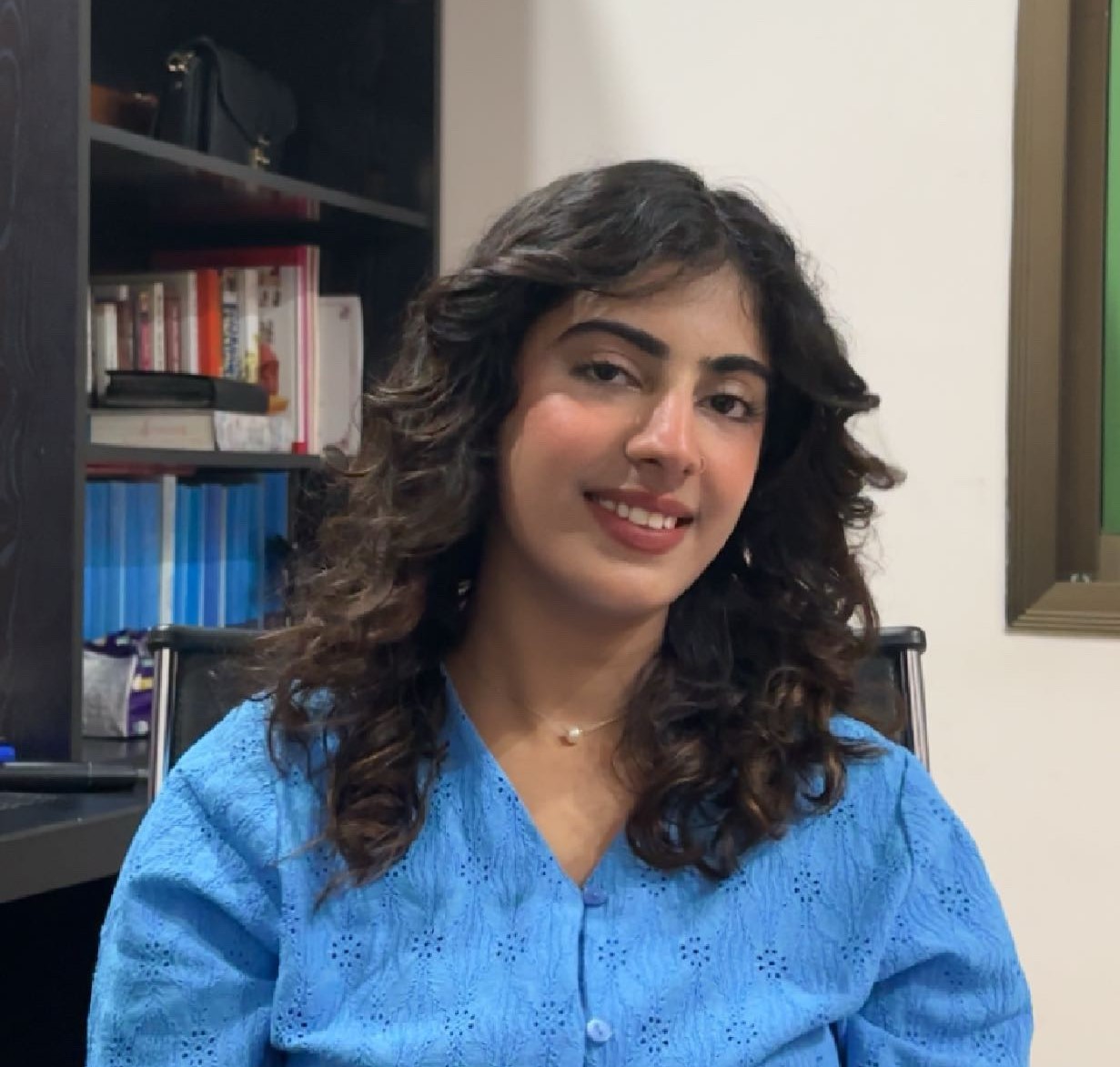
Manahil Samuel holds a Bachelor’s in Computer Science and has worked on artificial intelligence and computer vision She skillfully combines her technical expertise with digital marketing strategies, utilizing AI-driven insights for precise and impactful content. Her work embodies a distinctive fusion of technology and storytelling, exemplifying her keen grasp of contemporary AI market standards.