Folio3 AI Blog
Shaping Tomorrow with Intelligent Solutions
Explore the latest advancements in AI technology and how they're transforming industries for a smarter, more efficient future.
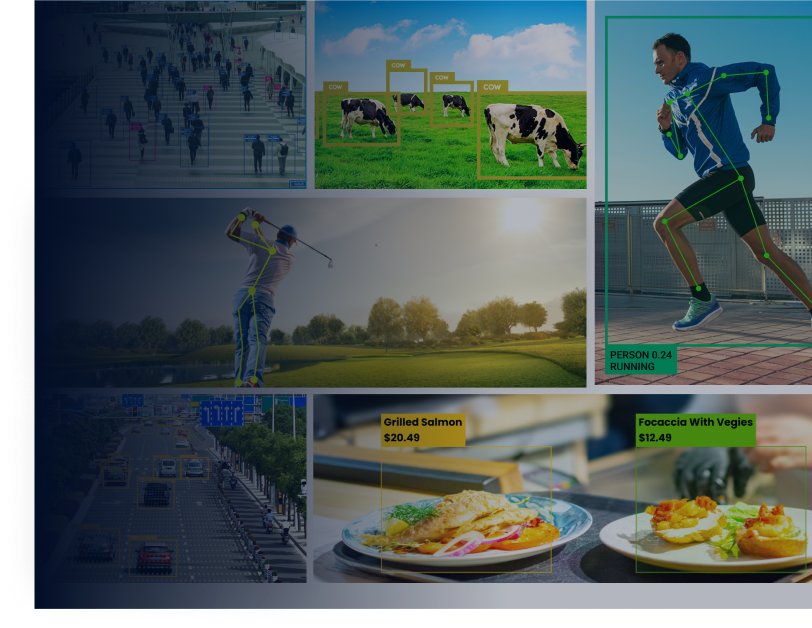
360 AI Insights with Folio3
Your hub for the newest AI developments, providing insights into state-of-the-art machine learning, transformative automation, and specialized AI applications. Explore expert opinions, success stories, and emerging trends that are pushing the boundaries of what's possible with artificial intelligence.
Converse Smartly
Sometimes, gathering our thoughts and writing a simple sentence is tough. Take our professional lives, for example. You have a brilliant idea for a project proposal and speak it confidentially in a meeting room, but when you sit down to document it, the words don’t flow. That’s what the best speech-to-text software is all about. […]
Author: Dawood Khan Barozai
Date: July 1, 2024
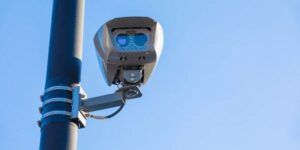
ALPR Smart City Solutions in the USA: Trends, Benefits & Adoption
American cities are quietly transforming their streets into intelligent networks. Automatic license plate recognition (ALPR) technology drives this change, turning ordinary traffic camer...
Read More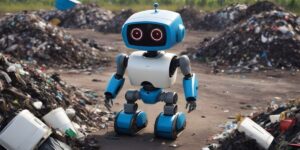
Manual vs AI Waste Management: What’s Better?
Waste management companies face the same daily struggle, trucks burning fuel to visit half-empty containers while other bins overflow and generate customer complaints. Drivers follow the...
Read More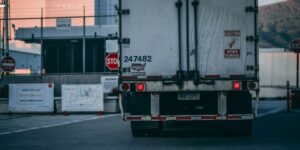
How to Use An Automated Weighbridge System To Boost Logistics Efficiency
Manual weighbridge operations create several challenges for businesses. These challenges include inaccurate weight records, slow processes, and costly breakdowns that disrupt logistics o...
Read More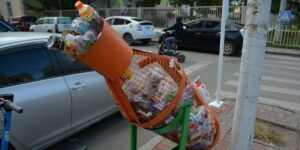
Smart Waste Management Solutions for Cities: Trends & Benefits
Most cities still collect waste in the same way they did decades ago, with fixed routes, scheduled pickups, and little insight into what happens between collection days. This outdated ap...
Read More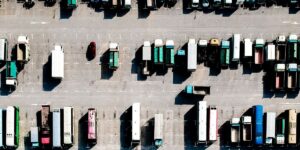
AI Vehicle Management Solutions to Cut Fleet Costs & Boost ROI
Fleet operations face mounting pressure from every direction. Rising fuel costs, unexpected breakdowns, and inefficient routes drain profitability daily. AI-driven vehicle management red...
Read More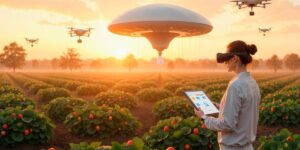
The Future of Food Security with Agriculture Drone Software
It is estimated that the world’s population will reach over 9 billion in the next 3 decades. That’s 2 billion more souls to share the already dwindling resources and 2 billion more mouth...
Read More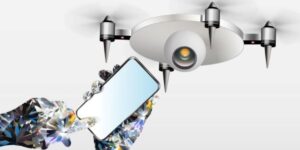
How AI Reduces Human Error in Drone Operations
Are you looking to implement technology that reduces human errors in your business? Not just you but many businesses across different industries are constantly looking for a tech solu...
Read More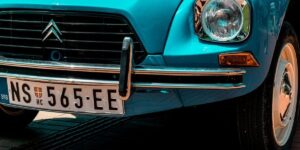
ALPR vs RFID: Key Differences, And Which One Is Right For you?
Vehicle identification technology has become essential for modern access control systems, with organizations across industries choosing between two primary solutions. RFID vs ALPR compar...
Read More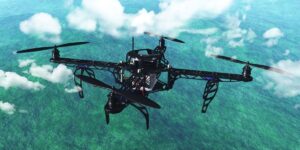
AI Shark Detection Drones: Use Cases & Benefits
AI shark detection drones are transfiguring marine safety for commercial fishing fleets, offshore platforms, and maritime operations by providing real-time threat assessment and crew pro...
Read More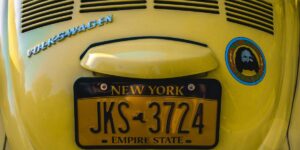
What is the Difference Between ALPR And ANPR?
ALPR and ANPR are two terms thrown around a lot in the license plate recognition world. Most people use them interchangeably, and they're mostly right, because both technologies do exact...
Read More