LLM agents, or Large Language Model agents, are AI systems that use large language models (LLMs) as their core engine to perform various tasks that require understanding and generating human-like text. These systems offer granular insights into complex questions by conducting in-depth analysis across past, present, and future data points.
Unlike simple questions that can be answered with a quick lookup, real-world problems often require multiple steps—some dependent on each other and others independent.
For example, answering “What’s the capital of France?” is a simple fact-based question. However, a more intricate question is: What were the main economic and political drivers behind France’s pension reform in 2023, and how did public sentiment evolve throughout the process? This requires a much more advanced approach.
In this case, the LLM agent must:
- Synthesize information across steps: Combine data, political commentary, and sentiment analysis to produce a coherent and insightful summary.
- Break down the question: Understand that it needs to provide an overview of economic and political factors, analyze specific events during the reform, and highlight shifts in public sentiment.
- Retrieve economic and political data: Access reports on policy changes, economic forecasts, and government statements.
- Analyze public sentiment: Use tools like social media analysis to track public opinion shifts over time, even consulting news archives and survey results.
Here, the agent’s ability to reason through interconnected topics, apply real-world knowledge, and use tools for data retrieval and sentiment analysis, allowing it to provide an insightful answer—far beyond a simple factual response.
This illustrates how LLM agents excel when faced with multi-step, interdisciplinary tasks that demand planning, memory, and specialized tools.
In essence, LLM agents go beyond simple responses. They reason through problems, plan solutions, and utilize specialized tools, making them capable of handling multi-step tasks efficiently.
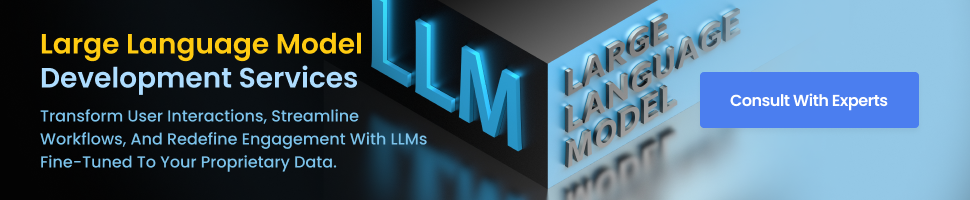
What are LLM Agents?
LLM agents are sophisticated AI systems that excel at handling complex tasks requiring step-by-step reasoning. They are trained on massive amounts of text data, and their iterative approach to solving complex problems makes them compatible.
These agents are not limited to answering straightforward questions—they can anticipate future needs, retain context from past interactions, and adjust their responses based on the task’s specific requirements.
“I believe that LLMs have the potential to become more general-purpose than any previous AI system. They could be used to solve a wide range of problems, from writing creative text to diagnosing diseases.”
Source: Geoffrey Hinton, interview with The New York Times, 2023.
For more understanding, let’s explore a basic scenario:
What are the tax rates for businesses operating in New York?
A general LLM with a retrieval-augmented generation (RAG) capability can quickly extract relevant information from tax databases.
Now, consider a more precise query:
How will upcoming changes in New York tax laws impact small businesses in the technology sector over the next two years?
This question demands more than retrieving facts. It involves interpreting new regulations, understanding their implications on different industries, and forecasting future business trends. A basic RAG system can pull relevant laws but may struggle to connect them with industry-specific insights or future challenges.
This is where LLM agents excel. They go beyond surface-level answers by breaking down complex questions into smaller, manageable tasks. In this case, the agent might:
- Retrieve the latest tax laws and identify upcoming changes.
- Analyze historical trends to see how similar businesses were affected by past changes.
- Summarize these findings and predict how future policies might influence the tech sector.
LLM agents require structured planning, memory to track progress across multiple steps, and the ability to access relevant tools and databases. These capabilities allow them to handle multi-step workflows efficiently, making them indispensable for solving complex real-world problems.
LLM Agent Components
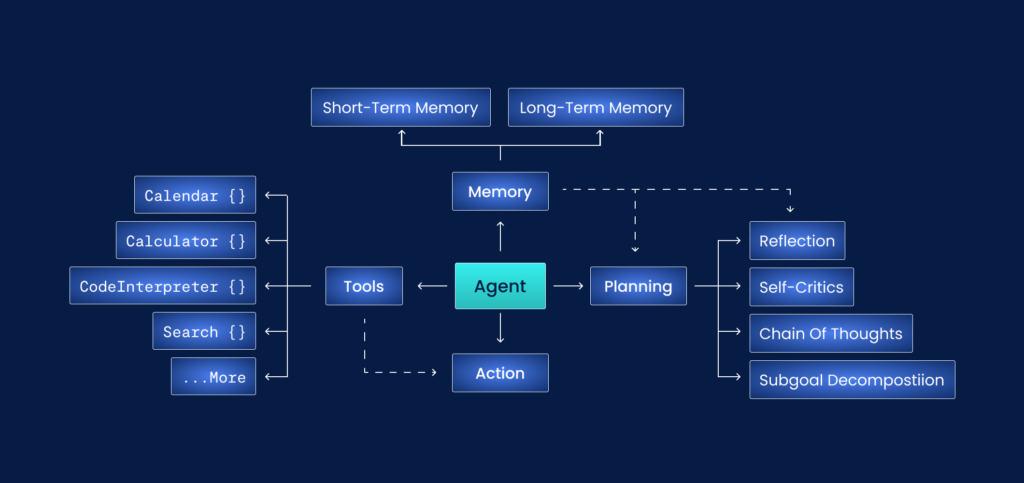
These components work together to form an effective LLM agent, making it capable of reasoning, planning, retaining context, and accessing the right tools to solve complex business scenarios.
- Agent/Brain: The agent serves as the “brain “of the system, using a large language model (LLM) to understand input, make decisions, and generate responses. It processes queries by reasoning through information, offering structured outputs instead of merely returning data.
Example: When asked, How can I increase engagement for a social media campaign? The agent not only pulls general tips but also suggests actionable steps, such as using interactive polls or scheduling posts for optimal audience activity times. - Planning: Planning enables the agent to break down complex problems into smaller, manageable tasks. The agent sequences these steps logically to reach the final solution without skipping essential details.
Example: For a query like, “How can I launch an e-commerce store in 30 days?” the agent creates a step-by-step plan:
Week 1: Market research and platform selection
Week 2: Product listing and website design
Week 3: Payment setup and logistics coordination
Week 4: Marketing campaign and launch preparation - Memory: Memory allows the agent to retain previous inputs, context, or steps taken during an ongoing session. This helps the agent maintain continuity in multi-step conversations and avoid redundant questions or errors.
Example:
Suppose a user asks in sequence:
“What’s the best time to visit Italy?”
“Can you find hotels in Rome during that period?”
The agent remembers that the user plans to visit Italy and narrows its search to Rome hotels for the given timeframe, making the interaction seamless. - Tool Use: Tool use refers to the agent’s ability to access external resources or specialized software to complete specific tasks. This could include APIs, calculators, or real-time databases to refine the accuracy and relevance of its responses.
Example:
When asked, “What’s Tesla’s latest stock price, and how does it compare with its performance last year?” the agent uses a financial API to fetch real-time data, cross-reference historical trends, and deliver a concise summary, offering deeper insights than a static response.
LLM Agent Frameworks
LLM (Large Language Model) agent frameworks provide tools and infrastructure to integrate language models (like GPT or Llama) into complex workflows, applications, and automation tasks. Here’s an overview of some prominent LLM agent frameworks.
1. LangChain
Overview: LangChain enables developers to build language-model-powered applications that combine data processing with LLMs, such as question-answering or summarization pipelines.
Key Features:
- Provides connectors to APIs, documents, and databases.
- supports multi-step reasoning and agentic behaviors
- has built-in support for OpenAI, Hugging Face, and other LLMs.
Use Case: Creating chatbots, knowledge-based applications, and multi-step workflows (e.g., generating insights from unstructured data).
2. Hugging Face Transformers
Overview: Although primarily a library for accessing LLMs, it also supports building intelligent agents using models from its vast hub.
Key Features:
- Access to thousands of pre-trained models
- APIs for model fine-tuning
- Support for building pipelines for text generation, classification, and more
Use Case: Deploying multi-modal applications combining NLP, vision, and audio models.
3. Haystack (deepest)
Overview: Haystack specializes in building agent-like applications focused on retrieval-augmented generation (RAG) to answer questions using knowledge bases.
Key Features:
- Integration with search engines like Elasticsearch and OpenSearch
- Tools for document indexing and QA
- Real-time data augmentation for queries
Use Case: Knowledge retrieval assistants that extract real-time information for customer support or research.
4. AutoGPT/BBabyAGI
Overview: AutoGPT and BabyAGI are experimental frameworks where LLMs act autonomously to perform tasks, iterating independently to reach goals.
Key Features:
- Task automation without manual intervention
- Agents capable of accessing the web and APIs
- Can chain tasks to self-improve
Use Case: Automating processes such as market research, competitive analysis, or task scheduling.
5. LlamaIndex (formerly GPT Index)
Overview: LlamaIndex is designed for creating agents capable of interacting with external data sources, including documents and APIs.
Key Features:
- Builds knowledge graphs from structured/unstructured data
- Works well with Llama-based models and other LLMs.
- Provides tools for custom queries and dynamic reasoning.
Use Case: Intelligent search applications using enterprise or proprietary data.
6. Streamlit + LLMs
Overview: While Streamlit is primarily a data app framework, it allows easy integration of LLMs to create interactive dashboards and smart assistants.
Key Features:
- Simple UI creation for agent-driven interactions
- Allows real-time text generation with integrated models
- Supports Python-based LLM frameworks
Use Case: Building user interfaces for summarization tools or interactive Q&A systems.
7. Dust.tt
Overview: Dust is a lightweight framework focused on creating autonomous agents that use external data sources and APIs dynamically.
Key Features:
- Focuses on integration with third-party tools (APIs, databases)
- Enables LLMs to act on instructions autonomously
- Tracks workflows and agent decision-making processes.
Use Case: Dynamic automation for business processes and API-based tools.
LLM Agent Challenges
Integrating LLM (large language model) agents into workflows or applications comes with several challenges. Below are the key obstacles faced by developers and businesses when building and deploying LLM agents:
- Hallucinations: LLMs sometimes generate factually incorrect or irrelevant responses to the query (“hallucinations”).
Challenge: Agents may confidently provide false or misleading information, critical in high-stakes environments like healthcare or finance.
Solution: Use retrieval-augmented generation (RAG) or restrict the agent’s outputs with knowledge-based constraints. - Limited Context Handling: Most LLMs can only handle a limited number of tokens (e.g., ~4k to 32k).
Challenge: This restricts their ability to handle long documents or complex multi-turn conversations.
Solution: Use tools like LlamaIndex to chunk data or summarization techniques to process large inputs incrementally. - Cost and Latency Issues: Running LLMs, especially large models, can be computationally expensive and slow.
Challenge: Real-time applications may experience lag, and costs can increase with frequent or heavy usage.
Solution: Optimize with smaller models (like Llama 2 or GPT-3.5) for certain tasks and only use large models for complex queries. Caching can also help minimize repeated calls. - Data Privacy and Security Risks: LLM agents often require access to sensitive data to perform tasks, which raises privacy concerns.
Challenge: It is critical to ensure compliance with privacy regulations (e.g., GDPR, HIPAA) and prevent data leakage.
Solution: Securely process sensitive data using on-premise LLMs or private APIs. Anonymization techniques can also protect user information. - Interpretability and Transparency: Understanding how the model arrives at certain outputs is difficult, especially in complex decision-making systems.
Challenge: Lack of explainability makes it hard to trust the agent’s actions, particularly in critical applications.
Solution: Implement explainable AI (XAI) frameworks and track the agent’s actions through logging and monitoring systems. - Ethical Concerns and Bias: LLMs may generate biased outputs or reinforce stereotypes based on the data they are trained on.
Challenge: Such biases can affect the fairness and inclusiveness of the agent’s responses.
Solution: Use bias detection tools and model fine-tuning to mitigate problematic outputs. - Autonomous Agent Control and Safety: Autonomous LLM agents (like AutoGPT) can make unexpected or undesired decisions without human oversight.
Challenge: Mismanagement of task chains or actions could cause unintended consequences.
Solution: Implement human-in-the-loop systems and limit the agent’s ability to take irreversible actions.
Types of LLM Agents
Here’s an overview of the different types of LLM (Large Language Model) agents, along with their characteristics and use cases:
1. Conversational Agents
Overview: Conversational agents, often referred to as chatbots or virtual assistants, are designed to engage in natural language dialogue with users. They can simulate human-like conversations and respond based on context.
Characteristics:
- Focus on understanding and generating human-like text.
- Capable of maintaining context over multiple turns
- Often include sentiment analysis to gauge user emotions.
Use Cases:
- Customer support chatbots that assist with inquiries
- Personal assistants like Siri or Google Assistant
- Social chatbots for entertainment and engagement
2. Task-oriented agents
Overview: Task-oriented agents are designed to accomplish specific tasks or workflows based on user input. They leverage LLMs to understand requests and provide relevant outputs or actions.
Characteristics:
- Focus on achieving defined objectives (e.g., booking a flight, scheduling appointments).
- Utilize external APIs and databases to perform tasks.
- capable of handling multi-step processes
Use Cases:
- Virtual booking assistants for travel and reservations
- Workflow automation tools in business applications
- Personal productivity tools that manage tasks and reminders
3. Creative Agents
Overview: Creative agents use LLMs to generate original content, such as text, poetry, stories, or artwork. They can assist in creative endeavors by providing inspiration or drafting pieces.
Characteristics:
- Focus on generating unique and imaginative outputs.
- Often employ different styles, tones, or genres based on user input
- Capable of iterative refinement based on feedback
Use Cases:
- Content creation tools for blogs, articles, or social media posts
- Story generators for writers and game developers
- Art generators that produce visual content based on textual descriptions
4. Collaborative Agents
Overview: Collaborative agents are designed to work alongside humans or other agents to enhance productivity and problem-solving. They can facilitate teamwork and communication in various environments.
Characteristics:
- Focus on teamwork and cooperation.
- capable of integrating with project management and communication tools
- Often, they adapt their behavior based on group dynamics.
Use Cases:
- Virtual brainstorming assistants that help teams generate ideas
- Co-writing tools that allow multiple users to contribute to a document
- Collaborative coding assistants that provide suggestions and help debug code
How FOLIO3 AI Helps Improve LLM Agents
FOLIO3 AI alleviates the functionality of large language model (LLM) agents by integrating advanced techniques in natural language processing, tailored datasets, and real-time adaptability.
This unique integration allows LLM agents to better understand context, generate more precise responses, and learn from user interactions dynamically.
The FOLIO3 AI LLM services also incorporate customizable modules that allow organizations to fine-tune LLM agents according to specific industry needs, ensuring more relevant and effective communication.
Additionally, it employs advanced analytics to continually improve performance based on user feedback, creating a feedback loop that enhances ongoing learning and interaction quality.
The Ending
Ultimately, each type of LLM agent serves distinct purposes, addressing different user needs and improving interactions in various domains.
Understanding these types helps in selecting the appropriate agent for specific applications, ultimately improving user experience and productivity.
These agents excel at engaging in meaningful conversations, grasping context, and producing responses that mimic human dialogue.
By adopting these innovative tools, we are stepping into a future where AI can quickly integrate into our routines, empowering users to tackle challenges with greater ease and efficiency.
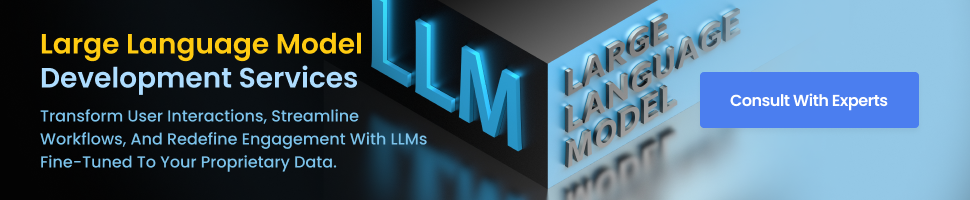
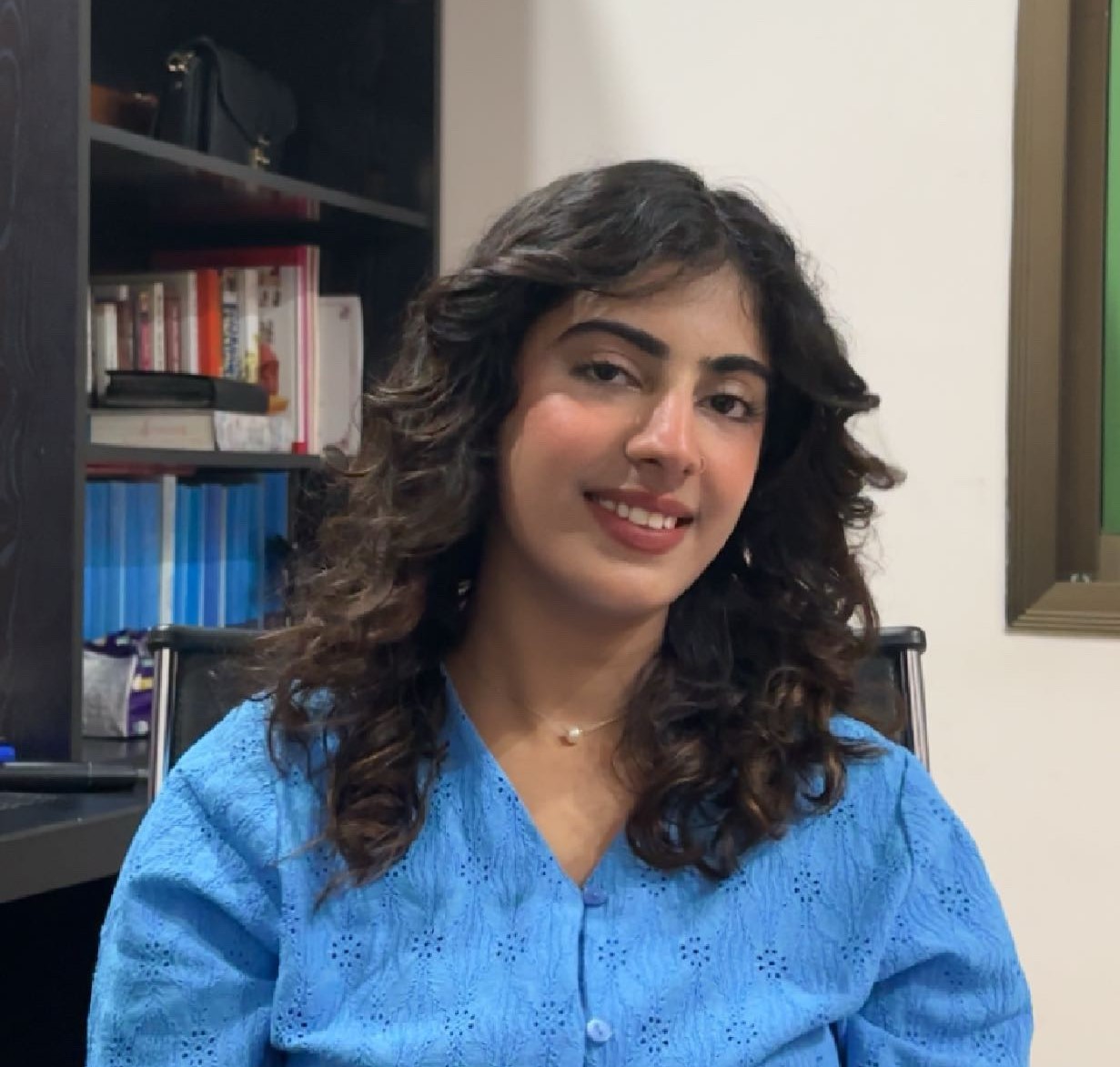
Manahil Samuel holds a Bachelor’s in Computer Science and has worked on artificial intelligence and computer vision She skillfully combines her technical expertise with digital marketing strategies, utilizing AI-driven insights for precise and impactful content. Her work embodies a distinctive fusion of technology and storytelling, exemplifying her keen grasp of contemporary AI market standards.