Executive Summary
In the world of artificial intelligence (AI), distinguishing between Generative AI vs. Large Language Models is essential for maximizing their potential. This blog aims to elucidate the disparities between Generative AI and LLMs, analyzing them across various facets such as definition, utility, applications, and collaborations.
Let us explore Generative AI vs. Large Language Models in-depth to gain a holistic understanding of their unique features and their potential impact. This exploration will give us the knowledge and insight necessary to make informed decisions.
Introduction
Artificial intelligence has witnessed remarkable progress, with Generative AI and Large Language Models emerging as pivotal technologies. While both share the goal of generating content, they differ significantly in their methodologies and applications. This exploration aims to clarify the attributes and functionalities of LLM Vs Generative AI and compare both approaches.
What Is Generative AI?
Generative AI encompasses a broad spectrum of AI technologies dedicated to producing new content across diverse formats, including text, images, and music. Leveraging techniques such as deep learning and probabilistic modeling, Generative AI services and solutions generate varied and lifelike outputs.
What Are Large Language Models?
Large Language Models (LLMs) are advanced AI systems trained on extensive datasets to comprehend and produce human-like text. Notable examples include OpenAI’s GPT series, renowned for their proficiency in tasks like language translation, summarization, and content generation.
Comparison: Generative AI vs. Large Language Models
By Definition:
Generative AI extends its purview beyond text generation, encompassing other creative domains like image synthesis and music composition. In contrast, Large Language Models specialize in processing and generating text-based content.
Example:
Generative AI Services: A generative AI system like DeepMind’s WaveNet can create realistic human-like speech, enabling applications such as voice assistants and interactive storytelling.
Large Language Models: OpenAI’s GPT-3 is a prime example of an LLM capable of generating coherent and contextually relevant text across various tasks, including text completion, question answering, and text summarization.
By Usefulness:
Generative AI services offer versatility across multiple modalities, catering to a wide array of creative endeavors. Conversely, Large Language Models excel in language-related tasks, providing sophisticated solutions in natural language processing.
Example:
Generative AI: Nvidia’s StyleGAN2 can generate high-resolution, photorealistic images of non-existent faces, revolutionizing the field of computer-generated imagery (CGI) and character design.
Large Language Models: GPT-3’s advanced language understanding capabilities make it invaluable for tasks like content generation, language translation, and sentiment analysis in natural language processing applications.
By Applications:
Generative AI finds applications in diverse fields such as image manipulation, artistic creation, and creative writing support. Large Language Models, on the other hand, are commonly utilized in language translation, chatbot development, and content generation for various platforms.
Example:
Generative AI: Google’s Magenta project leverages generative AI techniques to create music compositions and artworks, pushing the boundaries of creativity in the arts and entertainment industry.
Large Language Models: GPT-3 is widely used in various applications such as chatbots, content recommendation systems, and automated writing assistants, transforming how we interact with language-based technologies.
By Collaborations:
Generative AI technologies often collaborate with artists, designers, and musicians to produce innovative and engaging content. Meanwhile, Large Language Models collaborate with researchers, developers, and content creators to enhance language understanding and generate informative text.
Example:
Generative AI: The collaboration between Adobe and Nvidia resulted in the creation of GANPaint Studio, a tool that allows users to edit and manipulate images with incredible precision using generative AI algorithms.
Large Language Models: Companies like OpenAI collaborate with research institutions and industry partners to enhance LLM capabilities and develop innovative applications in natural language understanding and generation.
Select the Right Approach: LLM VS Generative AI
When faced with the decision between Generative AI vs. Large Language Models, it’s crucial to consider several factors to ensure you select the most suitable approach for your project. Here’s a breakdown of key considerations to help guide your decision-making process:
Type of Content:
Generative AI services excel in generating diverse content types beyond text, including images, music, and code. On the other hand, LLMs are tailored for text-based tasks such as natural language understanding, text generation, language translation, and textual analysis.
Data Availability:
Generative AI requires diverse datasets specific to the content type being generated. Conversely, LLMs are optimized to work with extensive text data, making them ideal for projects with abundant textual resources.
Task Complexity:
Generative AI is well-suited for complex, creative content generation tasks or scenarios requiring a variety of outputs. LLMs shine in tasks centered around language understanding and text generation, offering accurate and coherent responses.
Model Size and Resources:
Larger generative AI models demand significant computational resources and storage capacity. In comparison, LLMs may prove more efficient for text-focused tasks due to their specialization in language processing.
Training Data Quality:
High-quality and diverse training data are imperative for generative AI to produce meaningful outputs. LLMs rely on large, clean text corpora to effectively understand and generate language-based content.
Application Domain:
Generative AI finds its niche in creative fields like art, music, and content creation. LLMs, on the other hand, excel in natural language processing applications such as chatbots, content summarization, and language translation.
Development Expertise:
Developing and fine-tuning generative AI models can be challenging and requires expertise in machine learning and domain-specific knowledge. In contrast, LLMs, especially pre-trained models, are more accessible and user-friendly for text-based tasks, necessitating less specialized expertise.
Ethical and Privacy Considerations:
It’s crucial to consider ethical implications when utilizing AI models, particularly in scenarios involving sensitive content. LLMs are often fine-tuned to adhere to specific ethical guidelines, offering a level of control over model behavior.
Ultimately, the choice between Generative AI and LLMs should align with your project objectives, the nature of the content involved, and the available resources. In some cases, a hybrid approach combining both Generative AI and LLMs may offer the most comprehensive solution to address diverse project requirements.
Conclusion
In summary, while Generative AI vs. Large Language Models share the overarching objective of content generation, they diverge significantly in approach, capability, and application.
Understanding these distinctions is crucial for harnessing the right technology for specific tasks and domains. As AI continues to evolve, both Generative AI and Large Language Models will play pivotal roles in driving innovation and creativity across various industries.
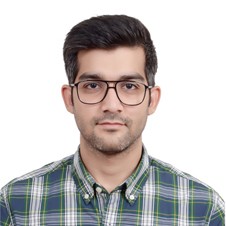
Dawood is a digital marketing pro and AI/ML enthusiast. His blogs on Folio3 AI are a blend of marketing and tech brilliance. Dawood’s knack for making AI engaging for users sets his content apart, offering a unique and insightful take on the dynamic intersection of marketing and cutting-edge technology.