Imagine a world where computer vision does not only interpret pixels but creates new realities from scratch. A world where machines don’t just see but dream, that’s the contribution of generative AI in computer vision!
Generative AI adds a realistic touch to images and videos, which helps systems learn better and become more accurate. This improves the quality of pictures, making them clearer. Not only that, but generative AI also creates 3D models from 2D images, which opens doors for virtual reality and robotics in many industries.
Generative AI and computer vision can together make any business creative, efficient, and cost-effective. It is used in many industries for a variety of purposes. In this guide, we will explore the applications and impact of generative AI services in computer vision.
Understanding Generative AI in Computer Vision
Generative AI in computer vision uses two neural networks—a generator and a discriminator. The generator creates images or videos that look real, while the discriminator’s role is to distinguish between real and fake images.
The two networks continuously compete with each other until the generator produces images that are similar to real ones. This whole process is known as training and is important because it’s how we get the super-realistic AI-generated images. The better the training, the more remarkable and authentic images it creates.
Role of Computer Vision in Modern Technologies
Computer vision has changed the way we interpret images. It has turned them into authentic visuals by bridging the gap between digital imagery and real-world applications. With the introduction of generative AI, this technology has become even more powerful. Whether it is for painting, designing, or creating human-like faces, generative AI can be integrated.
The integration of generative AI services in computer vision has opened up a new realm of possibilities in industries and our daily lives. It’s used in many different ways:
- At Work – It helps warehouse robots grab and sort items, guides driverless cars, and even helps in medical operations.
- In Health – Doctors and health practitioners use Generative AI to get a better look at medical scans, helping them catch diseases earlier and more accurately.
- For Safety – it’s behind facial recognition and unusual activity spotting in public places, helping keep areas like airports secure.
- For Shopping – It’s changing online shopping, suggesting products based on images, and letting you virtually try on clothes or makeup.
Looking ahead, computer vision has a lot more potential. It could transform self-driving cars, medical tests, and even how we create art pieces. However, it depends on how we use this technology. If used wisely, it can lead to a smarter, safer future.
Applications of Generative AI in Visual Understanding
Improving Image Recognition Using Generative AI
Generative AI improves image recognition, making it more accurate and dependable. These generated images are helpful for the system to learn and recognize objects in different contexts, lighting conditions, and orientations.
Moreover, generative AI also participates in augmenting low-quality images and making them clearer. This is particularly useful for security cameras or medical imaging, where image clarity is required. Here’s how it works:
Creating Additional and Superior Training Data
We know realistic images are important, but let’s understand it with an example. If you’re a detective with only a handful of blurry photos to catch your suspect. You wouldn’t be very good at recognizing faces now, would you?
However, with the help of best generative AI solutions companies and computer vision, you can get as many clear pictures as you need. It can even fill in missing parts of an image, which helps the image recognition system get better at identifying what’s in a picture, even under tricky conditions.
Beyond Mere Identification
The role of generative AI is not just to identify or name objects in a picture. But to make the system understand the whole scene, like how different things relate to each other. It can also get creative by changing the style of a picture or even creating completely new images from scratch.
Video Analysis and Synthesis using Generative AI
Video analysis and synthesis is another major application of generative AI in computer vision. It can create videos that look practical but are completely synthesized by the machine, which has improved the quality of live video feeds by deblurring or removing noise. Here is a concise overview:
Understanding Videos Better
Normally, figuring out what’s happening in a video, like spotting goals in a soccer match or catching odd things on a security camera, takes a lot of effort. But now, it is easy with generative AI because it can quickly scan through videos and understand actions, objects, and even how people feel.
Fixing and Changing Videos
Sometimes, security camera videos need a lot of fixing because they are damaged or some parts of the video are misplaced. Generative AI can assist in changing the video for better understanding and easy interpretation by filling in missing parts.
It’s important to consider that generative AI is used carefully to avoid any problems like creating fake videos that look real.
Advancements In Generative AI For Computer Vision
Technology continues to advance, and so will generative AI in computer vision. Here are some of the latest trends in this field:
Generative Adversarial Networks (GANs):
GANs excel in generating high-quality images and consist of two neural networks that compete with each other to produce more realistic images.
Hyper-realistic Face Generation:
It is possible to generate incredibly alike and convincing faces with computer vision AI applications using deep learning methods.
Style Transfer:
This technique transforms images into different styles, creating unique and artistic results. It is mainly used for personalized filters on social media apps and to recreate famous works of art.
Variational Autoencoders (VAEs):
VAEs are used for more specific and detailed images by learning the underlying structure of an image dataset. This technology lets fashion and interior industries generate new patterns and designs.
Improved Video Generation:
Generative AI services create realistic videos with complex motions and actions. It has great potential in industries like film and advertising, where creating visual effects can be time-consuming and costly.
Text-to-Image Generation:
This popular technology can generate images based on text descriptions, making it easier to create visuals for stories or articles. It has also been used in ecommerce to generate product images based on written descriptions.
Challenges and Ethical Considerations Of Generative AI In Computer Vision
Generative AI in computer vision has applications across the world. However, with so much creativity and AI-driven visual understanding, ethical considerations are inevitable. In this section, we will explore some of the major ethical challenges of generative AI for computer vision.
Bias in Data
One of the biggest challenge with generative AI in computer vision could be the biasness of data. The datasets used to train these systems are created by humans and can reflect their biases. It can particularly lead to biased decisions and predictions by the system, perpetuating existing societal biases.
Privacy Concerns
Another major ethical consideration in generative AI and computer vision is privacy concerns. The ability to generate realistic videos and images creates a potential for misuse and violation of privacy by creating fake images and videos. This poses a threat to media credibility and can lead to the spread of misinformation.
Misuse of Data/Technology
Generative AI and computer vision can be manipulated for unethical or illegal purposes, such as fake news or misuse of personal information. This highlights the need for responsible use of these technologies.
Accessibility and Inclusivity
Other ethical considerations in AI implementation are accessibility and inclusivity. If AI systems are created with biases or without considering the opinions of diverse populations, it can lead to exclusion and discrimination. It is important to ensure that these systems are accessible and inclusive for all.
Best Practices To Implement Generative AI In Computer Vision
To ensure the ethical and effective implementation of generative AI services and solutions in computer vision, here are some best practices and tips to remember:
Strategies for Integrating Generative AI into Computer Vision Systems
The key strategy for integrating generative AI into computer vision is to start with high-quality datasets and select appropriate algorithms, such as Generative Adversarial Networks (GANs) or Variationally Autoencoders (VAEs). This helps collect a diverse and comprehensive dataset to train the generative AI model that accurately represents and interprets the visual world.
Businesses also use a feedback loop where generated data informs and refines the computer vision system, creating a virtuous improvement cycle.
To further enhance the integration of generative AI into computer vision systems, businesses can also incorporate transfer learning techniques. This involves using pre-trained models and fine-tuning them for specific tasks or datasets.
Data Management and Quality Control
The quality of data produced holds more importance and authority in business operations, so it’s important to be open and transparent about the data, which may include human identification or vehicle plate information showing families’ backgrounds and addresses. Whatever decision you make must be clear—this builds trust with users and addresses privacy concerns.
Data management must be practiced from the beginning of the development process. It shows Generative AI applications are used for business success, not for fraud or illegal money investment.
Future Trends in Generative AI and Visual Technologies
Future trends in Generative AI and vision technology are always changing, and new ones are introduced. While all trends have their own importance, one important trend to inherit is the integration of generative AI with augmented reality (AR) and virtual reality (VR).
Another trend to watch is the integration of generative AI with natural language processing (NLP). This will allow for more advanced text-to-image generation, leading to better storytelling and creative content creation.
These immersive technologies can change industries and give us experiences that blend the physical and digital worlds.
Generative AI’s Role in Shaping the Future of Computer Vision
From learning platforms for education to virtual tours in real estate, computer vision with generative AI will change how we interact with our environment in 2024.
We will see generative AI transforming cameras into active participants that enhance images in real-time and even recreate missing details. Generative AI is also expected to transform an image into a digital form and perform certain operations to get useful information.
Beyond these applications, generative AI is confident in revamping how we gather and analyze data, making incomplete datasets complete. It’s speeding up the development of visual systems, ensuring they’re more efficient and reliable.
However, we need to be mindful of biases in AI-generated data and the privacy concerns of this technology. Ensuring ethical use and maintaining human oversight is vital.
Conclusion
Generative AI is a future where machines not only learn but understand human goals. It opens up exciting and unimaginable possibilities in our visual world.
The impact of generative AI development services in computer vision goes beyond just businesses; it influences society, culture, and ethics. It’s leading to big improvements in fields like healthcare, security, and entertainment, making tools more accessible to everyone and personalizing our digital experiences.
As with any technology, there are challenges that must be addressed, but if we do so with care and consideration, generative AI can change the way we interact with our visual world.
Frequently Asked Questions (FAQs)
How Does Generative AI Improve Computer Vision?
Generative AI improves computer vision by creating realistic images. It also helps machines understand visual data better, leading to more precise and efficient decision-making.
What Are the Main Challenges in Implementing Generative AI in Computer Vision?
The main challenges of generative AI in computer vision are related to data quality. Transparency is needed in data to achieve accurate and unbiased model outputs. These challenges can be addressed through proper planning, diverse team collaboration, and continuous system performance evaluation.
How Is Generative AI Changing Industry-Specific Applications?
Generative AI is changing industry-specific applications by making them reliable and accessible. For example, generative AI can generate synthetic images in healthcare to assist in diagnosis and treatment planning; in security, it’s used for facial recognition and object detection; in entertainment, it’s used to generate realistic CGI effects and personalized avatars.
What Ethical Concerns Surround Generative AI in Computer Vision?
Ethical concerns about generative AI in computer vision are data misuse, biases, and lack of transparency in decision-making processes.
What Future Trends Are Expected in This Field?
Future trends that we expect to see are the integration of generative AI augmented with virtual reality for immersive experiences and personalized content.
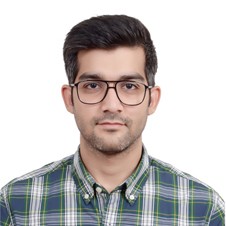
Dawood is a digital marketing pro and AI/ML enthusiast. His blogs on Folio3 AI are a blend of marketing and tech brilliance. Dawood’s knack for making AI engaging for users sets his content apart, offering a unique and insightful take on the dynamic intersection of marketing and cutting-edge technology.